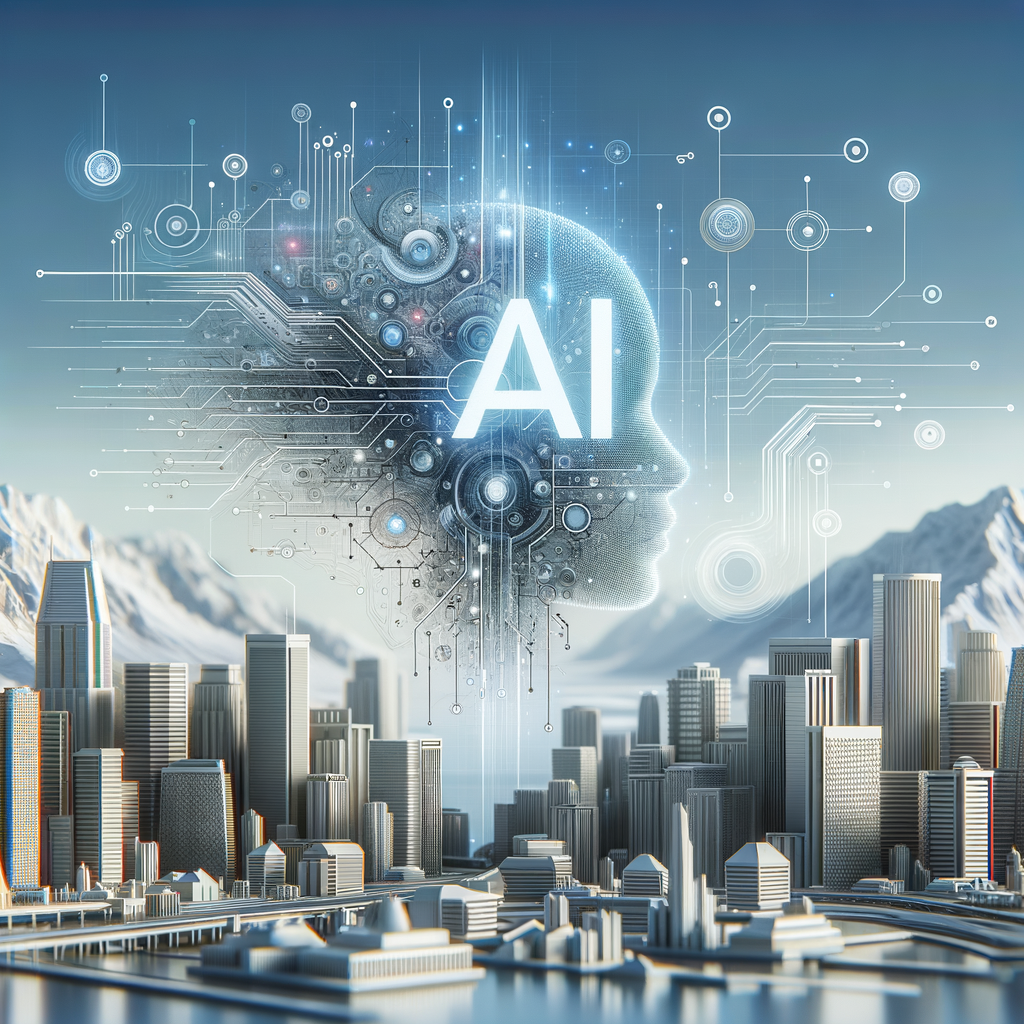
Venture into the AI Frontier: Exploring Autonomous Data Engineering
Dive into the evolving landscape of autonomous data engineering, where AI is reshaping how data is managed, processed, and utilized. This blog post will take you through the innovations and challenges in automating data workflows, enabling smarter, faster, and more efficient data practices. Discover how AI tools are transforming traditional data engineering roles and what the future holds in this exciting field.
Introduction
In today's rapidly evolving technological landscape, data engineering is undergoing a significant transformation. With the advent of artificial intelligence (AI) and machine learning (ML), we are witnessing a move towards autonomous data engineering—a paradigm shift designed to optimize and streamline the processes of data collection, processing, and management. This shift not only enhances the efficiency of how we handle data but also brings about a revolution in the roles and responsibilities of data engineers.
What is Autonomous Data Engineering?
Autonomous data engineering refers to the automation of data engineering tasks through AI and ML technologies. These tasks traditionally require significant manual effort, such as data cleaning, integration, and pipeline creation. By employing AI-driven tools, businesses can automate complex workflows, reduce errors, and speed up data processing, allowing for real-time analytics and decision-making.
The Role of AI in Data Engineering
AI plays a crucial role in redefining how data engineering tasks are performed. With advanced algorithms, AI can:
- Automate Data Cleaning: AI can automatically detect and correct errors in datasets, improving data quality and reducing the/manual intervention needed.
- Enhance Data Integration: AI-driven solutions can seamlessly integrate data from multiple disparate sources, ensuring consistency and accuracy.
- Optimize Data Pipelines: AI can predict optimal pathways for data flow, resulting in more efficient data processing and reduced latency.
Key Technologies Driving Autonomous Data Engineering
Several cutting-edge technologies are driving the move towards autonomous data engineering:
- AI-Driven ETL Tools: These tools leverage AI to extract, transform, and load data more efficiently than traditional methods.
- Machine Learning for Predictive Maintenance: ML algorithms identify potential bottlenecks in data pipelines before they impact performance.
- Natural Language Processing (NLP): NLP capabilities are enhancing user interaction with data platforms, allowing queries to be made in natural language.
Challenges and Considerations
Despite its many benefits, autonomous data engineering presents several challenges:
- Complexity of AI Models: Building and deploying AI models for data engineering tasks can be complex and require specialized expertise.
- Data Privacy and Security: As AI manages more data, ensuring data privacy and security becomes paramount.
- Integration with Legacy Systems: Legacy systems pose a significant challenge in implementing AI-driven autonomous solutions.
Future Implications
As AI continues to evolve, the future of data engineering looks promising. We can expect:
- Increased Adoption of AI Tools: More businesses will adopt AI-driven tools to remain competitive.
- Redefined Data Engineering Roles: New roles will emerge focusing on the supervision and fine-tuning of AI-driven processes.
- Towards a Fully Autonomous Architecture: The ultimate goal is a fully autonomous system where human intervention is minimal, allowing for incredibly fast and efficient data processing.
Conclusion
Autonomous data engineering is not just a trend—it is the future of how businesses will handle data. By embracing AI and ML technologies, companies can achieve unmatched levels of efficiency and innovation in data management. As these technologies continue to mature, the full potential of autonomous data engineering will become increasingly apparent, setting the stage for unprecedented advancements in the field.