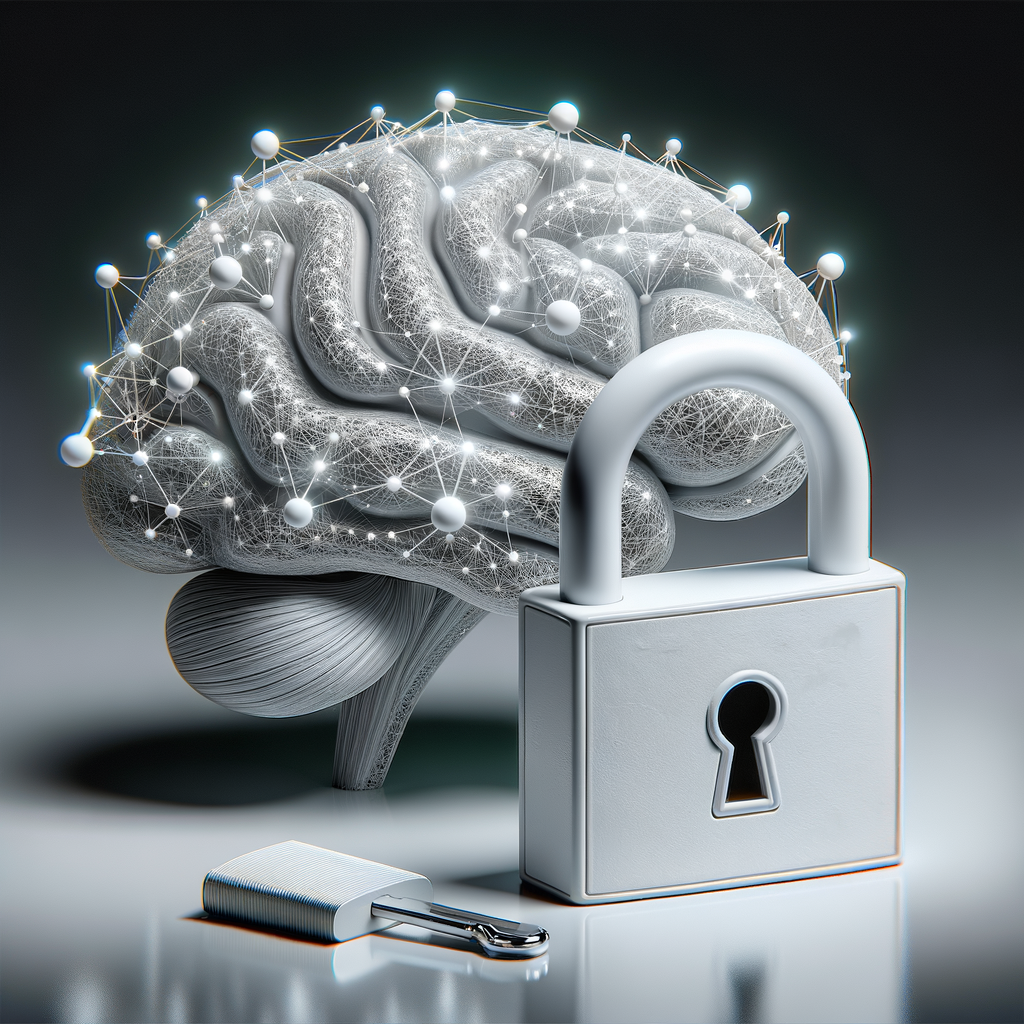
Unlocking the Future: The Intricacies of Neural Architecture Search in AI Development
Dive deep into the world of Neural Architecture Search (NAS) and discover how it's revolutionizing AI development. This blog explores the mechanisms, challenges, and breakthroughs in automating the design of neural networks to optimize performance and innovation in artificial intelligence.
Understanding Neural Architecture Search (NAS)
Neural Architecture Search (NAS) is a fantastical frontier in the field of artificial intelligence, where the design of the neural network architectures is automated. As the complexity of AI applications continues to rise, traditional manual design is increasingly seen as a bottleneck. NAS promises to overcome these limitations by providing a systematic way to explore the vast space of possible architectures, identifying the most efficient and effective designs far beyond human capability.
The Motivation Behind NAS
The motivation to engage in NAS comes from the desire to optimize deep learning models. Traditional approaches to designing neural networks involve expert-driven trial and error, which is not only time-consuming but often suboptimal. NAS can dramatically accelerate this process by using algorithms to iteratively evaluate and propose new architectures. This reduces both the time and expertise required for model development and leads to potentially better performing models.
Technologies Enabling NAS
Several technologies and methods underpinning NAS, including classic optimization techniques, reinforcement learning, evolutionary algorithms, and gradient-based methods. Each of these approaches has its strengths and weaknesses, and the choice often depends on the specific requirements of the task at hand.
-
Reinforcement Learning (RL): Using RL for NAS involves representing architectures as actions within a defined search space. An RL agent is then trained to identify the architectures that maximize some measure of performance. Examples include Google's AutoML.
-
Evolutionary Algorithms: These algorithms use principles of natural evolution, including selection, mutation, and crossover, to evolve architectures over successive generations. This approach has proven effective in various domains.
-
Gradient-Based Methods: Such methods focus on differentiable architecture search, which can significantly reduce the search time by leveraging gradients with respect to architecture parameters.
Challenges in NAS
Despite its potential, NAS faces several challenges:
-
Computational Resources: NAS is inherently computationally intensive. Evaluating a potentially performance-heavy architecture involves training it, which requires substantial computational resources.
-
Search Space Design: Designing a proper search space is critical for NAS success. Too broad a search space can lead to inefficiencies, while too narrow can constrain potential breakthroughs.
-
Scalability: As models become increasingly complex, scaling NAS methodologies to accommodate ever-larger search spaces and architectures is an ongoing challenge.
Breakthroughs and Future Directions
Recent advancements in NAS have shown promising directions, with a focus on making NAS more efficient and accessible. These include:
-
Transfer Learning: Leveraging knowledge gained from prior NAS tasks on new tasks can significantly reduce resource consumption.
-
One-Shot NAS: This technique involves training a super-network to predict the performance of child networks, reducing the need to fully train every candidate architecture.
-
Meta-Learning: Applying principles of meta-learning, where the learning algorithm learns to improve its own learning process across multiple tasks, to NAS shows potential in drastically improving efficiency and outcomes.
Conclusion
Neural Architecture Search represents a pivotal shift in how we think about designing AI systems. By automating the design of neural networks, NAS promises not only to enhance existing AI technologies but also to accelerate the pace of innovation across diverse fields. As the technology matures, it will increasingly become an integral part of AI development pipelines, unlocking unprecedented potential in creating smarter, faster, and more efficient AI models.