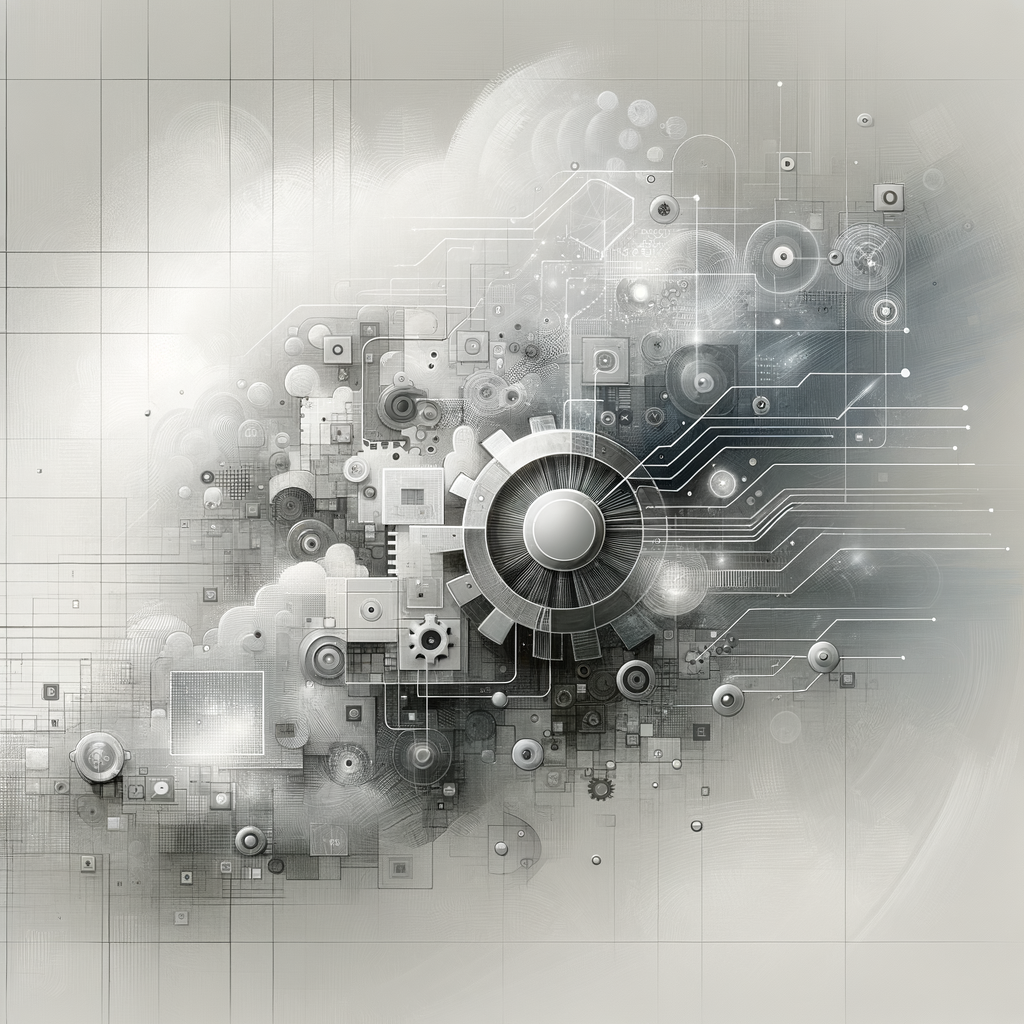
Unleashing the Power of Transformer Models: A New Era in AI and ML Innovation
This blog delves into the transformative potential of transformer models in AI and machine learning. Explore how these models are revolutionizing tasks such as language translation, text summarization, and more, while driving innovation across various industries. Discover the mechanics behind transformers, their impact on natural language processing, and the future prospects in AI advancements.
Unleashing the Power of Transformer Models: A New Era in AI and ML Innovation
Artificial Intelligence (AI) and Machine Learning (ML) have undergone significant transformations over the past few years, with one of the biggest game-changers being the development of transformer models. Introduced in the groundbreaking paper “Attention Is All You Need” by Vaswani et al. in 2017, transformers have since taken the world of AI by storm, becoming the foundation for powerful models like GPT, BERT, and many others.
In this blog, we will explore what transformer models are, how they work, and why they have become a pivotal element in AI and ML landscapes. We will also delve into their applications, benefits, and future potential.
What are Transformer Models?
Transformer models are a type of neural network architecture designed to handle sequential data with greater efficiency and effectiveness than previous models, such as Recurrent Neural Networks (RNNs) and Long Short-Term Memory (LSTM) networks. Unlike traditional models that process data in order, transformers employ a mechanism called self-attention, allowing them to consider the entire input data at once, which speeds up processing and improves accuracy.
One of the key innovations of transformer models is their ability to use attention mechanisms, which enhance the focus on relevant parts of an input for a given task. This attention mechanism enables transformers to capture long-range dependencies in data, making them exceptionally suited for tasks involving language and sequence understanding.
Key Components of Transformer Models
1. Self-Attention Mechanism
The self-attention mechanism is central to transformer models. It allows the model to weigh the importance of different words in a sentence, not just based on their sequential order but more on their contextual significance. This ability to draw global dependencies between inputs and outputs makes transformers highly efficient.
2. Encoder-Decoder Structure
Transformers are often built with an encoder-decoder structure. The encoder processes the input data, while the decoder generates the output. Each of these components is composed of layers that perform self-attention and feedforward operations, both crucial in generating meaningful representations of data.
3. Positional Encoding
Since transformers do not inherently understand the order of input sequences, they use positional encoding to retain positional information. This is crucial for language-based tasks where the order of words affects meaning.
Applications of Transformer Models
1. Natural Language Processing (NLP)
One of the most significant impacts of transformers has been on NLP. They power numerous applications, from language translation and sentiment analysis to question-answering systems and even chatbots.
Models like GPT (by OpenAI) and BERT (by Google) are built using transformer architecture and have set new benchmarks in NLP tasks, outperforming previous models in accuracy and speed.
2. Text Summarization
Transformers are exceptionally good at understanding context, making them ideal for generating concise summaries of large chunks of text while maintaining the core meaning and essential details.
3. Image Processing
While primarily used for text, transformers are starting to make waves in the field of image processing too, helping to classify image contents or enhance resolutions.
Benefits of Transformer Models
- Efficiency: They process data in parallel, significantly reducing the time required for training.
- Scalability: These models can be scaled to larger datasets with ease, leveraging vast amounts of data for better performance.
- Versatility: Apart from NLP, they are being adapted to various new challenges, such as music generation, programming code understanding, and more.
The Future of Transformer Models
The landscape for AI and ML is ever-evolving, and transformer models stand at the forefront. The future holds immense potential as these models are refined and adapted to handle even larger datasets and more complex tasks.
Innovations like the emergence of Sparse Transformers, which aim to reduce the computation cost by only attending to parts of the input data, suggest that the journey of transformer models is just beginning. As AI continues to evolve, transformers will likely play a crucial role in shaping its future.
Conclusion
Transformer models have revolutionized AI and ML with their elegant architecture and immense capacity to handle tasks that involve complex data patterns. As these models continue to evolve and integrate into various applications, they promise to unlock new possibilities in AI-driven solutions, spanning industries and enhancing human capabilities.
By harnessing the power of transformer models, we are on the brink of achieving monumental leaps in technology, reshaping everything from how we understand languages to how we interact with machines.