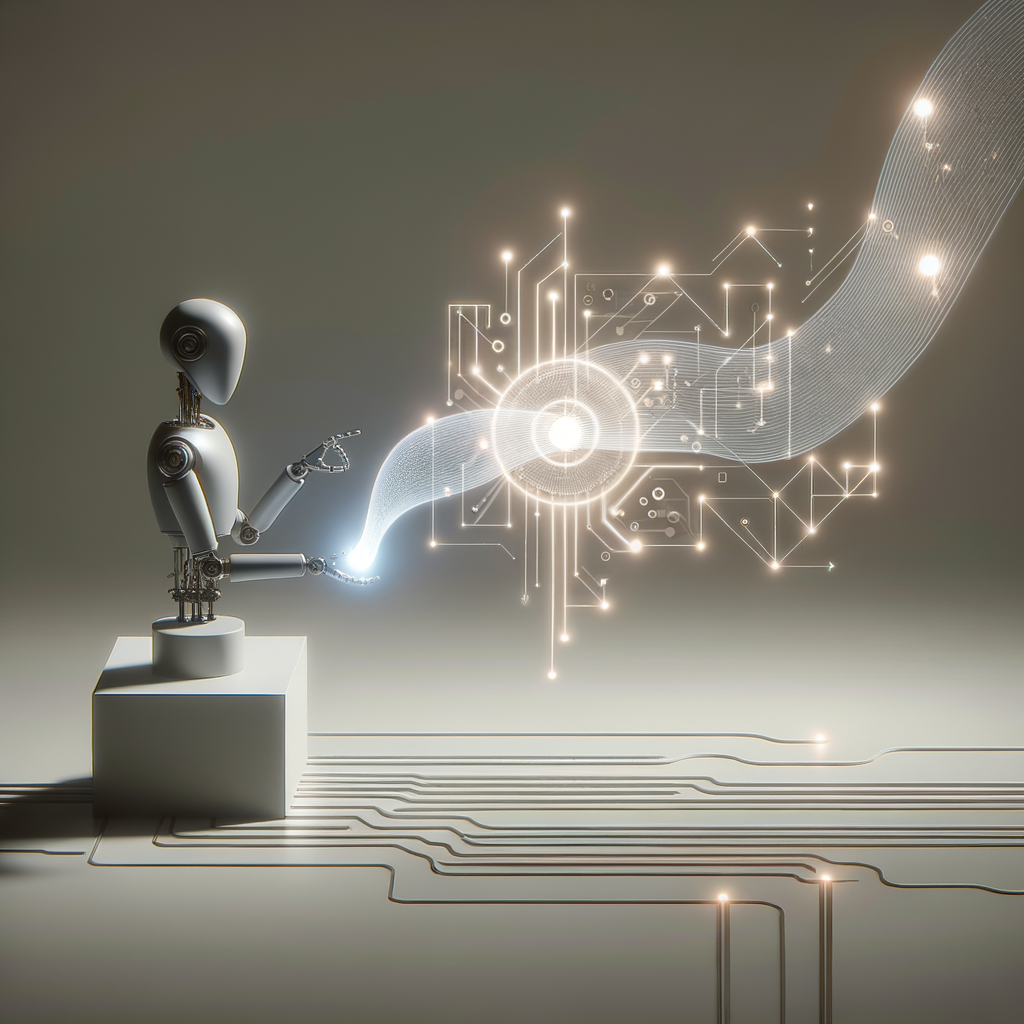
The Unseen Impact of Synthetic Data in AI Development
Discover how synthetic data is revolutionizing AI development by providing cost-effective, scalable, and privacy-conscious alternatives to real-world data. This blog delves into the generation, applications, and ethical considerations of synthetic data in machine learning and AI systems.
The Unseen Impact of Synthetic Data in AI Development
As artificial intelligence and machine learning continue to evolve, the need for quality data to train models becomes increasingly paramount. Traditional datasets, however, often come with a host of challenges—ranging from privacy concerns and limited availability to high acquisition costs. This is where synthetic data enters the picture. By simulating data that mimics real-world attributes without depending on actual personal information, synthetic data presents a promising frontier for AI development.
What is Synthetic Data?
Synthetic data is artificially generated information that can capture the statistical patterns and relationships present in real data. Unlike data anonymization techniques, which strip identifiable information out of datasets, synthetic data is created from scratch, ensuring that no actual user data is included.
How is Synthetic Data Created?
The generation of synthetic data involves using algorithms to observe patterns in existing datasets and then recreating similar, yet distinct, data points. This can involve simple statistical methods or advanced machine learning techniques like Generative Adversarial Networks (GANs).
Advantages of Synthetic Data
-
Privacy Protection: Since synthetic data does not contain any real user information, it significantly reduces the risk of privacy breaches.
-
Cost Efficiency: Obtaining real-world data can be prohibitively expensive. Synthetic data offers a cost-effective alternative, as it is generated programmatically.
-
Scalability: Synthetic datasets can be scaled up or down to meet the needs of different projects without the logistical challenges of real data collection.
-
Bias Reduction: By controlling the data generation process, developers can mitigate potential biases present in real-world data.
Applications of Synthetic Data
Synthetic data is finding applications across diverse fields including:
- Healthcare: To develop models without compromising patient privacy.
- Autonomous Vehicles: For simulating driving scenarios that are difficult or risky to capture in real life.
- Finance: To stress-test algorithms against a variety of market conditions.
Ethical and Practical Considerations
Despite its benefits, synthetic data is not without its challenges. One major consideration is ensuring that synthetic datasets accurately reflect the nuances of real-world data. Any significant disparity could lead to model errors or biases that can impact the performance of AI systems.
Furthermore, the ethics of synthetic data involve careful consideration. While it inherently supports privacy, the synthesized results still need vetting to ensure they do not introduce unforeseen biases or inaccuracies.
Conclusion
The use of synthetic data in AI development represents a powerful tool in overcoming the limitations of traditional datasets. Its capacity to safeguard privacy, cut costs, and provide scalable solutions makes it an increasingly attractive option for developers and researchers alike. As technology advances, the ways in which synthetic data can enhance AI models are bound to expand, offering deeper insights and more innovative applications.
Synthetic data stands as a testament to the ingenuity within AI—always finding new solutions to old problems. As we look to the future, understanding its role will be crucial for anyone invested in the field of artificial intelligence.