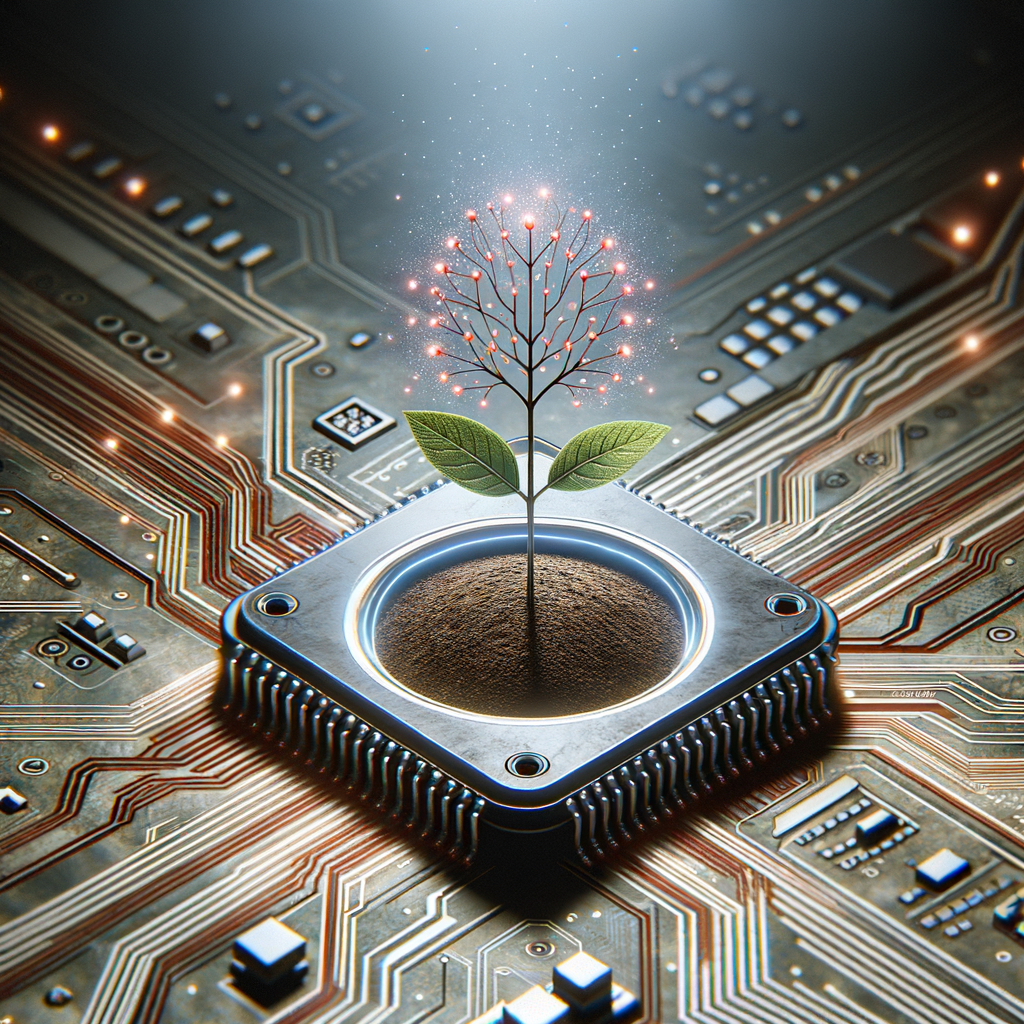
The Rise of Data-Centric AI: Transforming Machine Learning from the Ground Up
Explore the pivotal role that data-centric approaches play in the evolution of artificial intelligence and machine learning. This blog delves into how focusing on quality data rather than just models can redefine how AI systems are developed and deployed. We cover the principles, challenges, and future potential of data-centric AI, providing insights into its transformative power in creating more accurate and reliable AI solutions.
The Rise of Data-Centric AI: Transforming Machine Learning from the Ground Up
Artificial intelligence and machine learning have rapidly transformed how businesses operate and compete in today's digital landscape. Traditionally, the emphasis has been on developing sophisticated models, with the assumption that more complex models yield better results. However, there is a growing recognition that focusing on model-centric approaches has limitations. This has led to a paradigm shift towards data-centric AI, emphasizing the quality, quantity, and relevance of data as the cornerstone of successful AI systems.
Understanding Data-Centric AI
Data-centric AI refers to the practice of placing data at the forefront of AI and machine learning system development. This approach recognizes that inadequate, biased, or irrelevant data can severely affect the performance of even the most advanced models. Therefore, by refining the dataset itself—through techniques such as data cleaning, augmentation, and enriched annotation—models can achieve superior accuracy and reliability.
Why the Shift?
Several factors are driving this shift towards data-centric AI:
-
Data Quality Over Quantity: Unlike traditional models that relied heavily on volumes of data, data-centric AI focuses on the quality and integrity of the data.
-
Reduction of Bias: Model-centric approaches often fall short in reducing biases, largely because the datasets used are inherently biased. A data-centric approach enables practitioners to scrutinize datasets to minimize bias and enhance fairness.
-
Efficiency and Scalability: Focusing on data improves efficiency, as models trained on high-quality data require fewer computational resources and time.
-
Adaptability and Robustness: By enhancing datasets, models become more adaptable to real-world applications and improve in robustness.
Key Principles of Data-Centric AI
The transition to a data-centric approach requires adherence to several vital principles:
-
Prioritizing Data Quality: Uphold rigorous data hygiene practices by eliminating noise and ensuring data completeness and validity.
-
Domain-Specific Annotation: Ensure that data labeling is carried out with detailed accuracy, often requiring domain-specific knowledge to enhance the richness of datasets.
-
Data Augmentation and Synthesis: Employ techniques to augment datasets within ethical boundaries to ensure diversity and comprehensiveness.
-
Continuous Improvement Cycle: Develop feedback loops that allow continuous evaluation and improvement of datasets based on model performance.
Implementation Challenges
Despite its advantages, implementing data-centric AI poses certain challenges:
-
Resource Intensiveness: Gathering, cleaning, and maintaining vast amounts of high-quality data can be resource-intensive.
-
Data Privacy and Security: With greater scrutiny over datasets, ensuring privacy and data protection is paramount.
-
Skill Gaps: Shifting focus requires retraining existing teams or hiring skilled professionals who specialize in data science and domain expertise.
Future Potential
The future of AI is increasingly data-centric. This approach not only holds the potential to dismantle barriers that currently limit AI utilization but also paves the way for more democratized and equitable AI systems in various industries.
As companies begin to integrate data-centric practices, they must strive for a seamless alignment of data engineering and AI development teams. With their combined expertise, organizations can harness the full potential of their data assets, leading to breakthrough innovations and truly transformative solutions.
In conclusion, while efficient model design remains essential, the emphasis on the data-centric approach in AI marks a significant evolutionary step. By prioritizing data quality and enrichment, we can achieve more trustworthy and impactful AI implementations, ushering in new possibilities for innovation and progress.