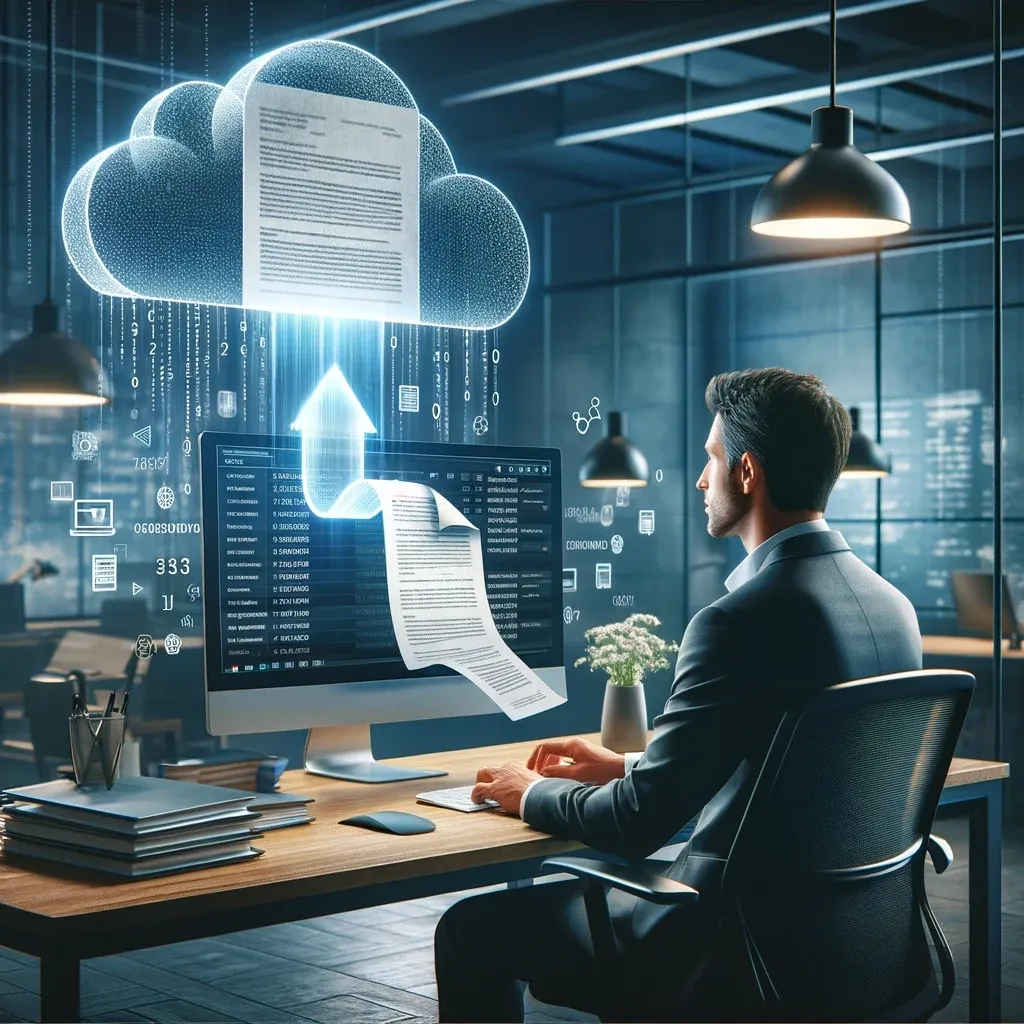
The Power of Retrieval-Augmented Generation with LangChain
In the rapidly evolving landscape of artificial intelligence, one of the most exciting developments is the advent of Retrieval-Augmented Generation (RAG). This technology represents a leap forward in AI's ability to process, understand, and generate human-like text. Powered by LangChain, a library designed to supercharge applications with large language models (LLMs), RAG is setting the stage for a new era of AI-driven applications that can interact with information in more sophisticated and useful ways.
What is Retrieval-Augmented Generation?
Retrieval-Augmented Generation is a cutting-edge technique that combines the power of language models with external knowledge sources to enhance the quality and relevance of generated text. RAG works by first retrieving information related to a given query from a vast dataset and then using this information to inform the generation process. The result is an AI that can produce responses that are not only coherent and contextually appropriate but also deeply informed by real-world knowledge.
The Role of LangChain in Harnessing RAG
LangChain, developed as a framework to facilitate the creation of applications leveraging LLMs, plays a pivotal role in implementing RAG. It provides developers with the tools and protocols needed to integrate retrieval systems seamlessly with language models. LangChain's architecture is designed to be flexible and adaptable, allowing for customization based on the specific needs of an application, whether it’s for summarizing content, answering questions, or generating entirely new insights.
The Impact of RAG on AI Applications
The implications of Retrieval-Augmented Generation for AI applications are profound. By leveraging RAG, AI can achieve a level of understanding and contextual awareness previously unattainable. This opens up new possibilities across various domains:
- Customer Support: AI can provide more accurate and detailed responses to customer inquiries by accessing a company’s knowledge base in real-time.
- Content Creation: Writers and content creators can use AI to generate articles, reports, and stories that are not only creative but also deeply researched.
- Education and Research: AI can aid students and researchers by quickly retrieving and synthesizing information from a plethora of sources.
Navigating the Challenges
While RAG and LangChain present significant opportunities, they also introduce challenges. The accuracy of the retrieved information is paramount, requiring robust datasets and effective filtering mechanisms to ensure reliability. Additionally, integrating RAG into existing systems poses technical challenges that require a deep understanding of both the technology and the specific application domain.
Looking Ahead: The Future Powered by RAG
As we look to the future, the potential of Retrieval-Augmented Generation to transform AI applications is immense. With tools like LangChain lowering the barrier to entry, we are on the cusp of a new era where AI can interact with human knowledge in unprecedented ways. The journey ahead is not without its hurdles, but the promise of more intelligent, informed, and useful AI applications is a compelling vision that drives the field forward.
In conclusion, the fusion of RAG and LangChain is not just a technological advancement; it's a paradigm shift in how we envision the role of AI in our digital lives. As we continue to explore and expand the boundaries of what AI can achieve, the synergy between retrieval mechanisms and generative models will undoubtedly play a central role in shaping the future of artificial intelligence.