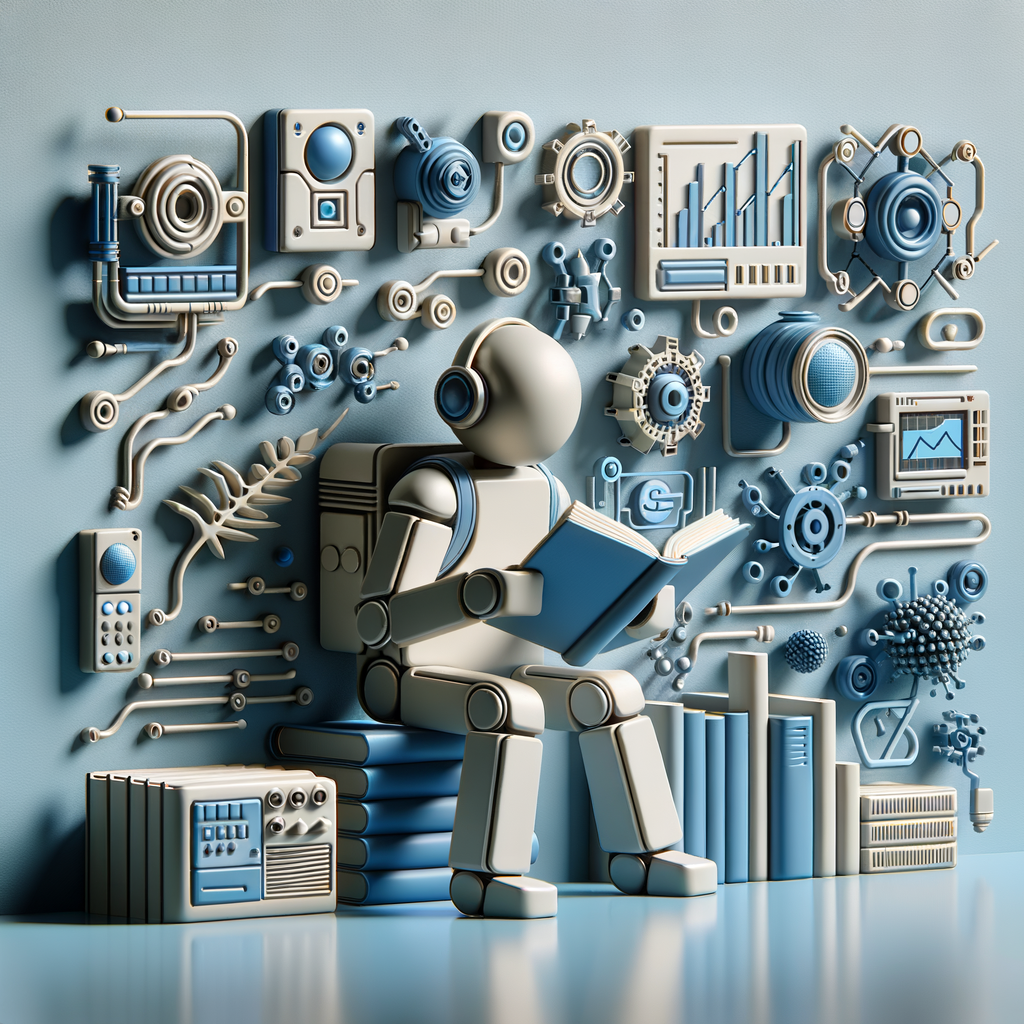
The Next Frontier: Crafting Zero-Shot Learning Models with AI
Delve into the realm of zero-shot learning models in AI and discover how these systems enable tasks without prior specific data training. Understand the technology, its applications, and the future possibilities it opens in AI advancements.
The Next Frontier: Crafting Zero-Shot Learning Models with AI
Artificial Intelligence (AI) has revolutionized countless industries with its capacity to learn and execute tasks. While traditional models rely heavily on vast amounts of labeled data for training, zero-shot learning (ZSL) takes a remarkably groundbreaking approach. This blog post explores the paradigm of zero-shot learning, its applications, advantages, challenges, and its future in the AI domain.
What is Zero-Shot Learning?
Zero-shot learning is an innovative machine learning approach that allows models to predict unseen classes without any specific examples to learn from during the training phase. It functions by leveraging semantic knowledge and connections between known and unknown classes, enabling the model to make inferences about new tasks effectively.
Core Principles of Zero-Shot Learning
-
Semantic Embedding: ZSL leverages a bridge built between the input space and output label space. Semantic representations (like word vectors) facilitate these connections, offering a rich relational context.
-
Transfer Learning Foundation: By harnessing features learned from familiar domains or tasks, ZSL models can apply this foundational knowledge to novel categories or tasks.
-
Knowledge Graphs Utilization: Semantic networks like WordNet can provide essential relational data, enriching the model's capability to understand relationships between unfamiliar inputs and its known counterparts.
Applications of Zero-Shot Learning
1. Image Recognition
Zero-shot learning can identify and label new objects in images without requiring re-training on a separate data set. This is crucial in scenarios like real-time wildlife tracking or security where species or objects may not have existing large datasets.
2. Natural Language Processing (NLP)
In NLP, zero-shot models are adept at understanding new languages or dialects with no direct prior exposure. They are particularly valuable in language translation tools.
3. Robotics
In robotics, ZSL enhances the flexibility and adaptability of machines, enabling them to interact with unfamiliar objects or environments without prior specific programming.
The Advantages of Zero-Shot Learning
- Scalability: ZSL models save significant resources by reducing the need for extensive labeled datasets for every new class or task.
- Adaptability & Flexibility: Capable of learning and functioning across tasks without re-training makes ZSL ideal for dynamic environments.
- Cost-Effective: Minimizing data preparation and labeling makes ZSL a more affordable approach.
Challenges Faced by Zero-Shot Learning
Limited Accuracy
ZSL models are often less accurate than traditional models, especially when the semantic space is not robustly built or lacks relational integrity.
Knowledge Representation Complexity
Building comprehensive and precise semantic networks is challenging and can lead to incorrect predictions if not adequately developed.
Contextual Limitations
ZSL models can misunderstand context since they aren't explicitly trained to consider every potential scenario or variation.
Paving the Way Forward
Zero-shot learning holds great promise for the future of AI, aligning with growing needs for scalability and adaptability in AI systems. Continued advancements in semantic embeddings, comprehensive relational databases, and innovative learning algorithms will further strengthen ZSL applications.
Future Directions
- Integrating More Robust Semantic Frameworks: To improve model accuracy and contextual understanding.
- Combining with Reinforcement Learning: To potentially boost learning adaptability and correct dynamically through interaction data.
- Focus on Real-world Applications: Expanding beyond academic proofs to direct industry implementations will showcase ZSL's utility and drive innovation.
Conclusion
Zero-shot learning represents a thrilling chapter in AI's evolution, echoing promises of greater adaptability and efficiency. As research continues and technology advances, ZSL may soon become a mainstay in diverse AI applications, pushing the boundaries of what AI can accomplish without exhaustive learning requirements.