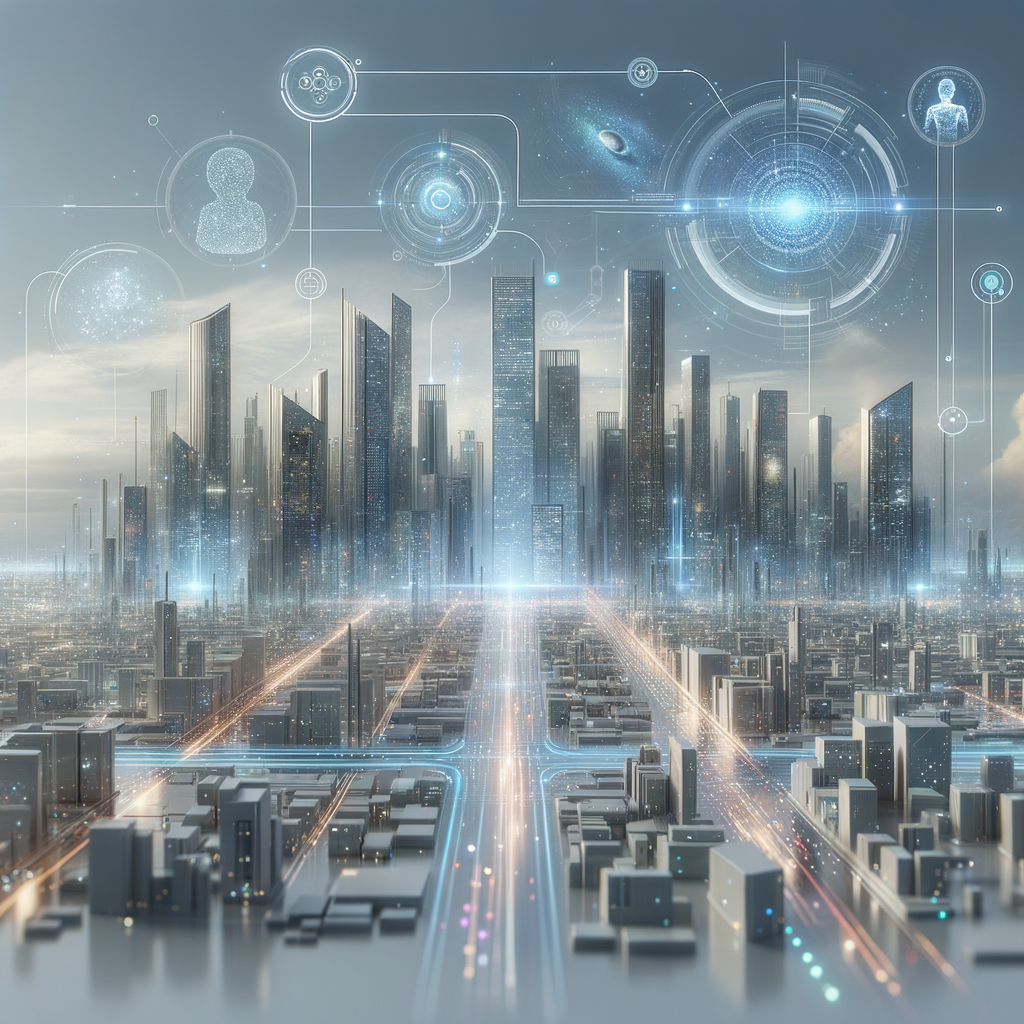
Revolutionary AI Architectures: Building Tomorrow’s Intelligent Systems
Explore the transformative impact of cutting-edge AI architectures on the evolution of intelligent systems. This blog post delves into how novel architectures like Transformers are redefining AI capabilities, enhancing both efficiency and performance in AI-driven applications. Discover the principles behind these advancements and their implications for the future of artificial intelligence.
Revolutionary AI Architectures: Building Tomorrow’s Intelligent Systems
Artificial Intelligence (AI) is constantly evolving, boasting innovations that increasingly push the boundaries of what machines can achieve. At the heart of these advancements are AI architectures - the frameworks that dictate how these systems learn, process, and interact with data. In this blog post, we'll explore some of the most revolutionary AI architectures that are shaping the future of intelligent systems.
Understanding AI Architectures
Before delving into specific architectures, it's crucial to understand what constitutes an AI architecture. Essentially, an AI architecture is a blueprint for the structure of an AI system, outlining how data is inputted, processed, and outputted. It orchestrates how algorithms and models come together to form a cohesive AI system.
Key Components of AI Architectures
- Input Layer: Where data enters the system.
- Hidden Layers: Intermediate processing stages where data transformation occurs.
- Output Layer: Where processed data, results, or predictions are produced.
Transformative AI Architectures
Recent strides in AI have been heavily influenced by new architectures that enable more sophisticated data handling and decision-making.
Transformers: The Game Changer
Initially developed for natural language processing, transformer models, such as GPT by OpenAI, have revolutionized how machines understand and generate human languages. Transformers employ a mechanism known as 'attention' which allows the model to consider the context of an entire sentence rather than processing word-by-word. This capability enhances the model's understanding and generation of contextually accurate responses.
How Transformers Work:
- Self-Attention Mechanism: Empowers the model to weigh the importance of different words in the input sequence.
- Parallelization: Unlike RNNs, transformers can process data more parallelly, accelerating training times.
Convolutional Neural Networks (CNNs) for Vision
Convolutional Neural Networks (CNNs) have been the backbone of most vision-based AI applications. From image recognition to video analysis, CNNs have continually demonstrated their efficacy.
Key Elements of CNNs:
- Convolutional Layers: Extract spatial and temporal features from images.
- Pooling Layers: Reduce the dimensionality of features, improving computation efficiency and reducing overfitting.
Graph Neural Networks (GNNs): The Next Frontier
As data becomes increasingly interconnected, the ability of AI to navigate complex relationships is crucial. GNNs offer a powerful means to process data structured as graphs, such as social networks or molecular structures in chemistry.
GNN Attribute Highlights:
- Node Analysis: Examines individual nodes deeply within a graph structure.
- Edge Analysis: Understands connections and relationships between nodes.
The Impact of Novel AI Architectures
The progression in AI architectures holds significant implications for a multitude of sectors:
- Healthcare: Enhanced model precision could improve diagnostic techniques and patient care.
- Financial Services: Data-driven insights can bolster risk management and customer personalization.
- Transportation: Intelligent systems could lead to more efficient and safer autonomous driving technology.
Challenges and Future Directions
Despite the tremendous potential of these architectures, substantial challenges remain. Scalability, data privacy, and ethical considerations are paramount. To address these concerns, ongoing research is essential, focusing on optimization and robust AI systems that prioritize human-centric values.
Looking Ahead
The future of AI lies in developing architectures that are flexible, interpretable, and aligned with human intent. The journey is as exciting as the destination, with cutting-edge research promising to continue reshaping our technological landscape.
In conclusion, as we dive deeper into AI architecture innovation, it's vital to acknowledge how these frameworks bring theoretical research closer to practical application, creating sophisticated systems that truly understand and interact seamlessly with their environments.