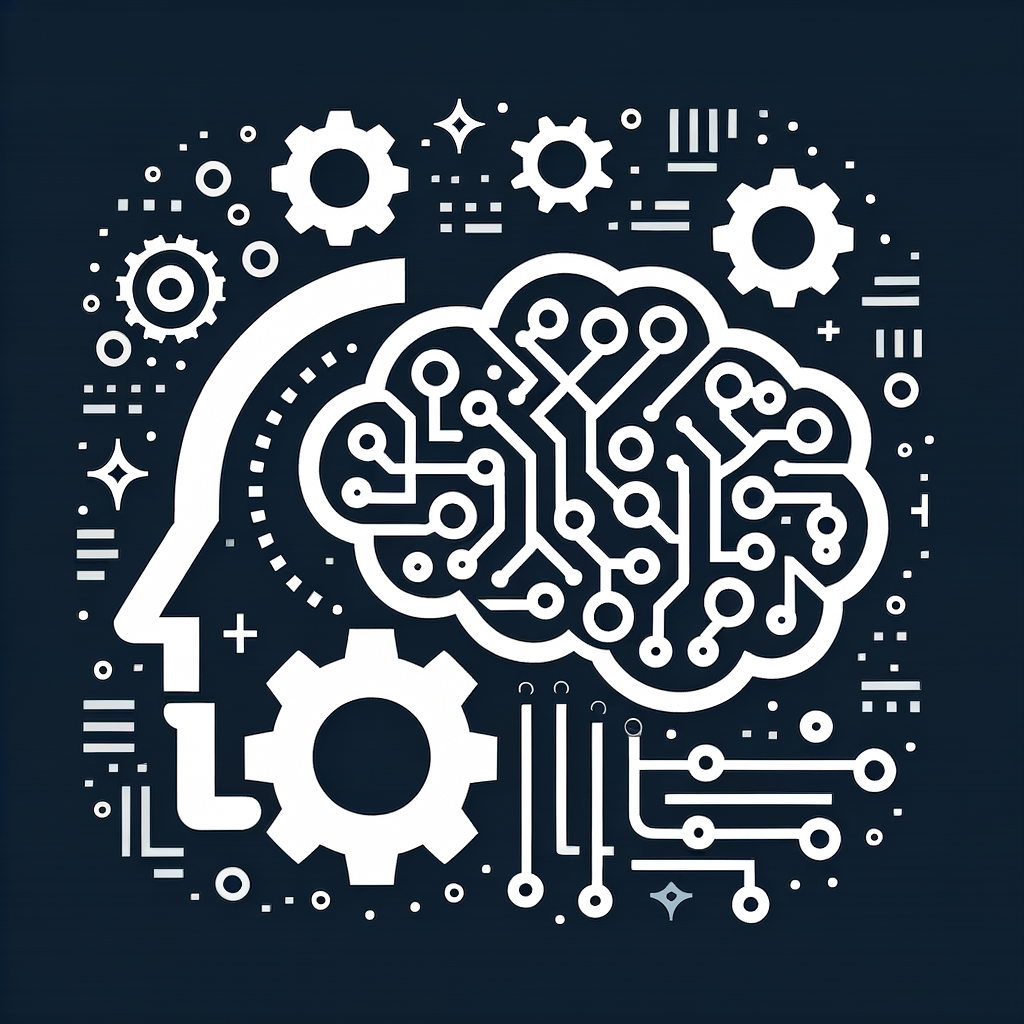
Leveraging AutoML: Simplifying Machine Learning for Everyone
Explore the world of Automated Machine Learning (AutoML) and discover how it is democratizing machine learning, making it accessible to developers and non-experts alike. This blog delves into the principles of AutoML, its benefits, and how it is transforming industries by simplifying the creation and deployment of machine learning models.
Leveraging AutoML: Simplifying Machine Learning for Everyone
As technology continues to evolve, the demand for machine learning applications has grown exponentially across various industries. However, building effective machine learning models traditionally required significant expertise in data science, statistics, and programming. The advent of Automated Machine Learning (AutoML) is revolutionizing this landscape by democratizing access to machine learning tools and enabling even non-expert users to develop and deploy models efficiently.
Understanding AutoML
Automated Machine Learning, or AutoML, refers to the process of automating the end-to-end process of applying machine learning to real-world problems. AutoML solutions simplify the process of model selection, hyperparameter tuning, data preprocessing, and feature engineering, thus reducing the manual effort required in the machine learning pipeline.
The Core Components of AutoML
-
Data Preprocessing: Automatically cleaning and preparing raw data is crucial for effective model learning. AutoML tools handle missing values, categorical data encoding, and outlier detection without manual intervention.
-
Feature Selection and Engineering: AutoML systems can automatically select the most relevant features from the data and even create new features by combining existing ones, enhancing the predictive performance of machine learning models.
-
Model Selection: By evaluating multiple algorithms and determining which one performs best for the task at hand, AutoML eliminates the need for users to possess extensive knowledge of various machine learning algorithms.
-
Hyperparameter Optimization: Finding the optimal hyperparameters is often time-consuming. AutoML employs strategies like grid search and random search, often augmented by more advanced techniques like Bayesian optimization, to fine-tune model parameters for superior performance.
-
Model Evaluation and Deployment: Once a model is trained, AutoML tools assess its performance using unseen data. They provide metrics like accuracy, precision, recall, and F1 score, helping users decide if the model is ready for deployment.
Advantages of AutoML
- Accessibility: AutoML opens the doors for those without a deep understanding of machine learning to leverage ML capabilities effectively.
- Efficiency: By automating repetitive tasks, it significantly reduces the time and effort required to develop models, enabling quicker iterations.
- Scalability: With AutoML, deploying machine learning at scale becomes more feasible as it reduces the complexity involved in maintaining and updating numerous models.
- Consistency: Automated processes reduce the room for human error, leading to consistent results across different datasets and tasks.
Transforming Industries Through AutoML
AutoML is not just a tool but a transformative force. From finance to healthcare, industries are adopting AutoML to drive innovation. In finance, AutoML is utilized for fraud detection, risk management, and algorithmic trading. Healthcare sectors are leveraging it to enhance diagnostic accuracy and tailor personalized treatment plans.
Challenges and Considerations
While AutoML offers immense potential, it also poses challenges. The black-box nature of automated models can lead to a lack of transparency, making it difficult for users to understand how decisions are being made. Furthermore, ethical considerations must be addressed to ensure fairness and accountability in automated systems.
The Future of AutoML
The future of AutoML holds exciting possibilities. As technology advances, the personalization of AutoML tools and the integration of explainable AI (XAI) methodologies are anticipated. These developments will address current limitations, increasing trust and improving the efficacy of machine learning applications across various domains.
Conclusion
AutoML is significantly lowering the barriers to entry for machine learning, inviting a broader range of users to engage with this powerful technology. By automating complex tasks, it empowers organizations and individuals to innovate faster and solve complex problems with less effort. As AutoML continues to evolve, it is poised to reshape the landscape of machine learning, making it an integral tool for the future.