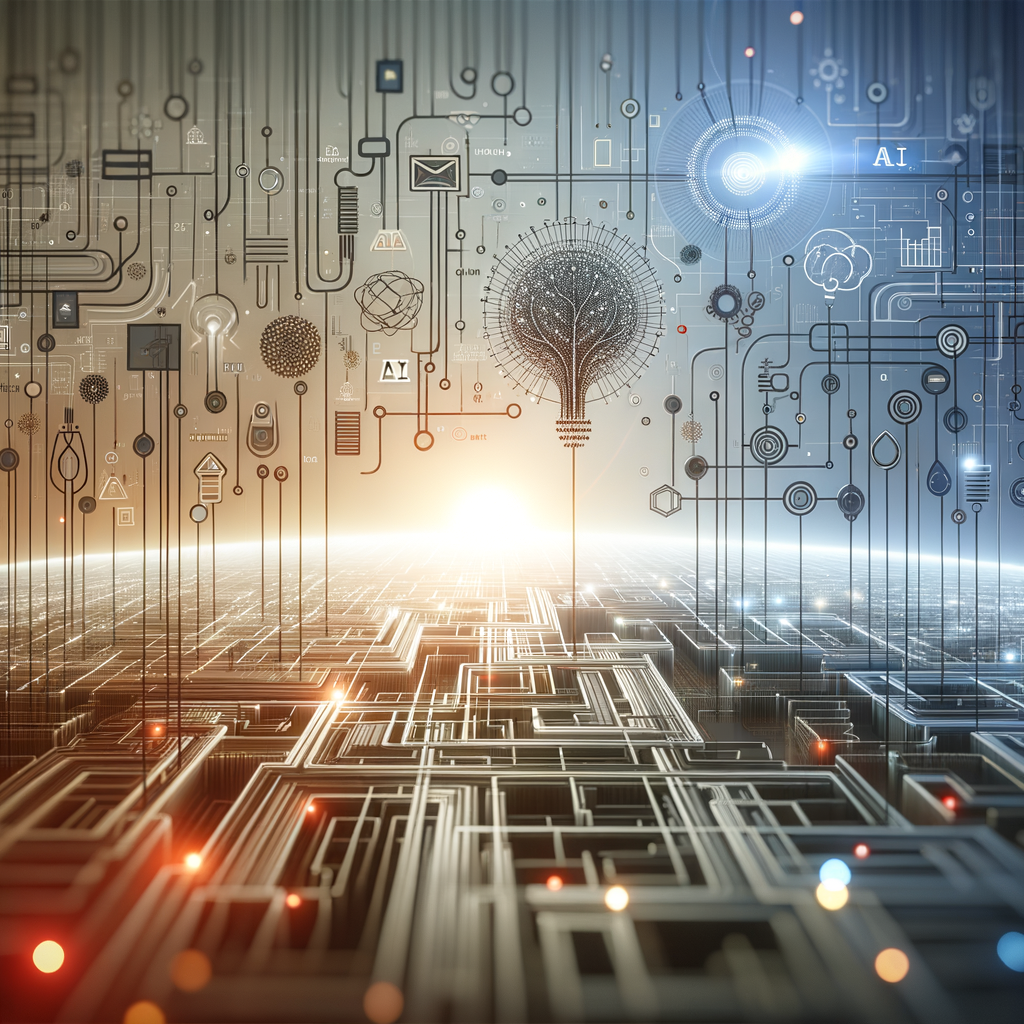
Innovative Horizons: Rethinking Data Engineering with AI
Explore the transformative impact of AI on data engineering. This blog delves into how artificial intelligence is revolutionizing data collection, analysis, and management. Learn about cutting-edge tools and methodologies reshaping the field, enhancing efficiency, and opening new avenues for innovation.
Introduction
In recent years, the fusion of artificial intelligence (AI) with data engineering has ushered in a transformative era in technology. By harnessing the capabilities of AI, data engineering is evolving beyond its traditional confines, embracing new possibilities that pave the way for smarter and more efficient data operations.
The Role of AI in Data Engineering
Automating Data Collection
AI algorithms can automate the collection of vast amounts of data, significantly reducing the time and effort required for data engineers. With the help of AI-powered tools, data can be scraped, cleansed, and organized at an unprecedented scale and speed, allowing data engineers to focus on more complex analytical tasks.
Enhancing Data Analysis
Machine learning models can identify patterns, correlations, and anomalies within datasets with high precision. This capability enables data engineers to derive meaningful insights quickly, improving decision-making processes across various industries. AI-driven data analysis helps in predictive analytics, offering foresight into future trends and behaviors.
Improving Data Management
AI tools facilitate optimized data storage and retrieval, ensuring fast and reliable access to information. By implementing intelligent indexing and query optimization techniques, data engineers can drive efficiency, especially for big data environments.
Tools and Methodologies
Emerging AI Tools for Data Engineering
- AI-driven ETL Tools: Modern ETL (Extract, Transform, Load) tools equipped with AI algorithms streamline data operations, ensuring accuracy and speed.
- DataOps: Utilizing AI in DataOps automates data pipeline processes, promoting a culture of continuous integration and delivery in data-centric applications.
Methodologies
- AI-Powered Data Governance: By leveraging AI, organizations can implement more effective data governance strategies, ensuring compliance and data security.
- Continuous Learning Systems: The integration of machine learning models into data pipelines allows for continuous improvement in data analytics capabilities.
Challenges and Considerations
While the benefits are substantial, the integration of AI in data engineering is not devoid of challenges. Data privacy concerns, algorithm bias, and the need for skilled personnel to manage AI systems are significant hurdles that need addressing.
Future Directions
The future of data engineering is intricately linked with advancements in AI technology. Innovations in AI, such as reinforcement learning and AI-enhanced cybersecurity measures, will likely dictate the next steps in data engineering evolution.
Conclusion
As AI continues to break new ground, its impact on data engineering is profound and far-reaching. Embracing these changes not only promises increased efficiency and innovation but also poses an exciting frontier for data engineers committed to driving the future of technology.
With AI at the helm, data engineering is poised for growth, pushing the boundaries of what is possible in data analytics and management. As we continue to explore these technologies, the possibilities are endless, setting the stage for a new era of data-driven insights and decisions.