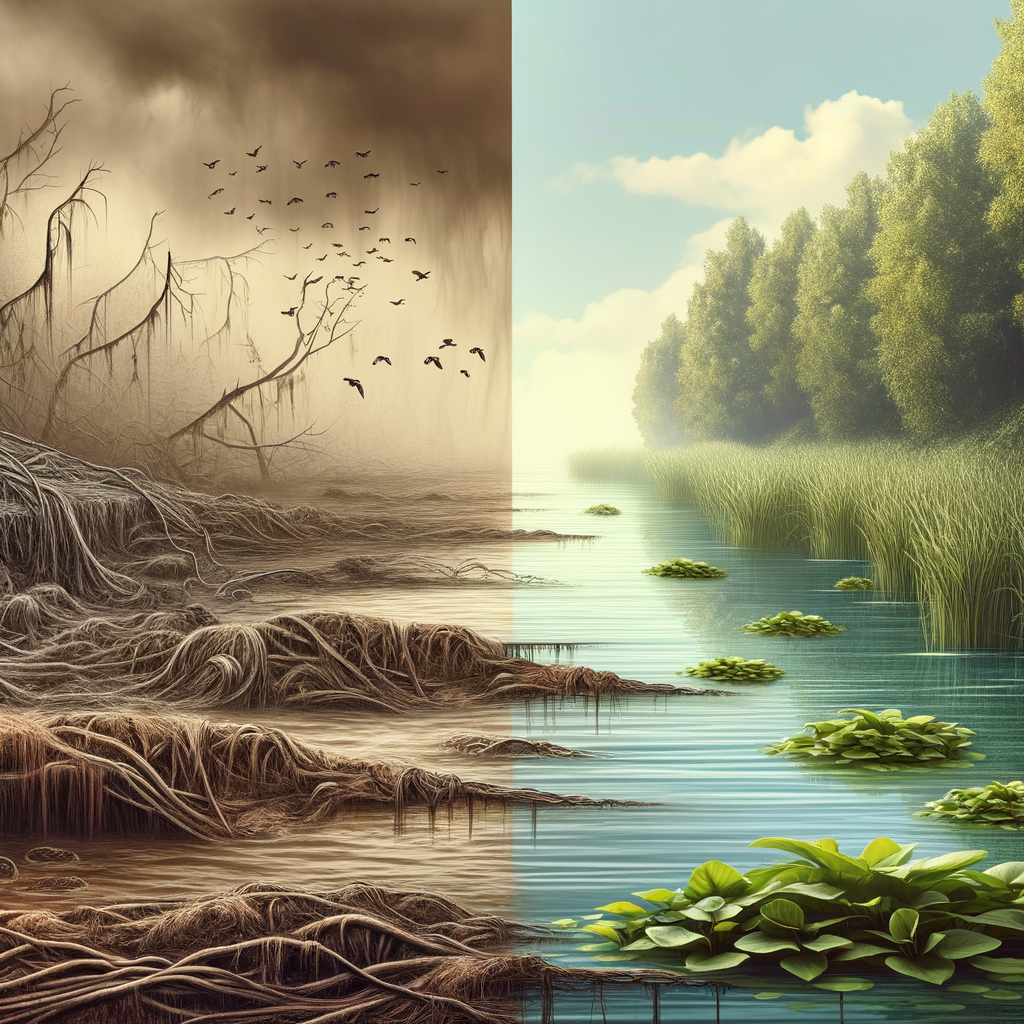
From Data Swamps to Data Lakes: Engineering Solutions for Efficient Data Management
Explore innovative strategies to transform data swamps into data lakes using advanced data engineering techniques. This blog delves into methodologies, tools, and best practices for managing data efficiently, ensuring accuracy and accessibility in today's data-intensive world.
Introduction to Data Swamps and Data Lakes
In the increasingly data-driven world, businesses generate and store vast amounts of data. However, not all data storage solutions are created equal. Two terms often coined in data storage discourse are 'data swamp' and 'data lake'. While a 'data lake' represents a structured, organized form of storage, a 'data swamp' implies unstructured, neglected data that can hinder business needs.
What is a Data Swamp?
A data swamp is essentially a deteriorated data lake. It stems from the mismanagement of data over time, causing the repository to become inefficient and unreliable. Reasons for this transformation from lake to swamp include lack of governance, absence of metadata, insufficient data quality practices, and uncontrolled data access.
When a data lake is not properly maintained, it becomes a swamp—messy, disorganized, and ineffective for analytical use. Identifying a data swamp involves recognizing attributes such as inconsistent data formats, poor data quality, and data that lacks context or categorization.
Defining a Data Lake
In contrast, a data lake is a centralized repository that allows you to store all your structured and unstructured data at any scale. You can store your data as-is, without having to first structure it, and run different types of analytics—from dashboards and visualizations to big data processing, real-time analytics, and machine learning—to guide better decisions.
Engineering Solutions for Data Swamps
Data Governance
The first step in converting a data swamp into a data lake involves implementing strong data governance policies. Data governance ensures that data is accurate, available, and usable. Establish governance frameworks that define data ownership, quality standards, and access controls.
Utilizing Metadata
Metadata management is crucial for finding and utilizing data efficiently. Good metadata practices involve maintaining a data dictionary, consistently updating data lineage records, and encouraging self-service data discovery. Metadata enables users to understand the data they are dealing with and facilitates effective data search and retrieval.
Data Quality Management
Regularly assess and cleanse the data to maintain high levels of data quality. Implement data validation scripts, profiling, and cleansing tools to identify and rectify data anomalies to prevent the accumulation of unreliable data.
Access Management
Apply strict access controls to ensure that only authorized users can view or edit data. Role-based access control (RBAC) can provide a structured approach to managing user permissions, enhancing security while sustaining data utility.
Automation Tools
Automation tools play a pivotal role in maintaining a healthy data lake. Employ data cataloging tools to consistently update and manage your metadata. Automation reduces human error, ensures consistency, and enhances data engineering efficiency.
Conclusion
Transitioning from a data swamp to a data lake requires a comprehensive strategy involving governance, quality management, automation, and robust metadata practices. As companies continue to store massive datasets, effective data management becomes pivotal to deriving actionable insights and gaining competitive advantages.
By implementing these engineering solutions, organizations can transform chaotic and inefficient data swamps into organized, efficient data lakes that support valuable analytics and decision-making processes.