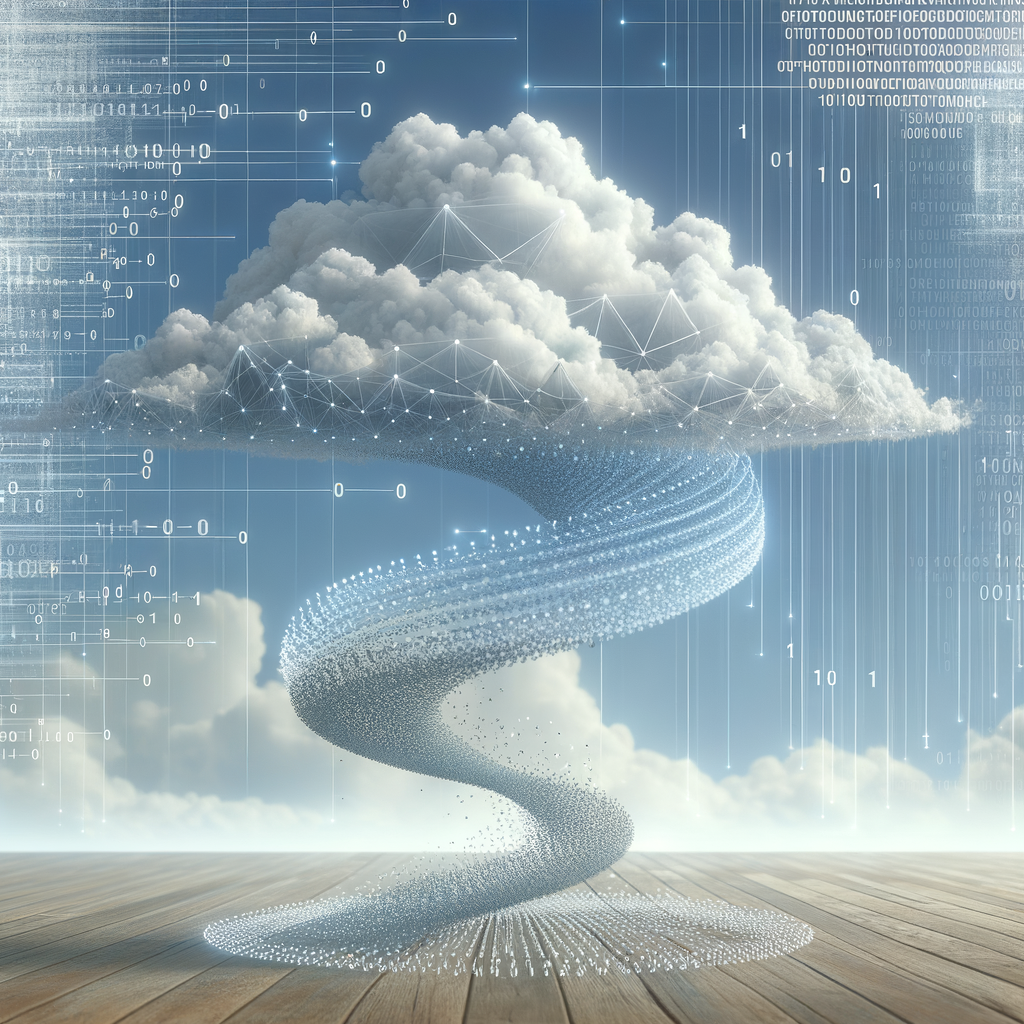
From Code to Cloud: Navigating the Future of Distributed AI Systems
Explore the transformative journey of deploying AI systems from local environments to the expansive cloud. This blog delves into the nuances of cloud-based AI, examining both technical challenges and innovative solutions that are propelling the future of distributed artificial intelligence.
From Code to Cloud: Navigating the Future of Distributed AI Systems
Artificial Intelligence (AI) has transitioned from an emerging trend to a crucial component of modern technological solutions. As AI models become increasingly complex, the need for powerful computing resources is undeniable. One major trend addressing this need is the movement from on-premises computing to cloud-based AI solutions. This blog explores the transition, detailing how distributed AI systems are the future of efficient and scalable AI deployment.
The Rise of Cloud Computing in AI
The advent of cloud computing has transformed various sectors by offering scalable, reliable, and cost-effective solutions. For AI, the cloud provides a platform that supports extensive computation without the limitations of local hardware. This flexibility allows businesses and researchers to innovate and experiment with larger models.
Benefits of Cloud-Based AI
-
Scalability: Unlike on-premises solutions, cloud services provide resources on demand. This ensures that AI applications can handle varying loads efficiently.
-
Cost Efficiency: With cloud computing, organizations pay only for the resources they use. This is particularly beneficial for startups and small enterprises needing AI capabilities without the upfront hardware investment.
-
Accessibility: Cloud platforms enable AI models to be accessed and updated remotely. Developers worldwide can collaborate seamlessly, contributing to faster iterations and improvements.
-
Security: While security concerns exist with data in the cloud, modern cloud providers offer robust security measures, ensuring that sensitive information and models stay protected.
-
Integration: The cloud offers integration capabilities with various other technologies like IoT, enhancing the ability of AI solutions to interact with diverse systems.
Key Technologies Driving Cloud-Based AI
As AI integrates more with cloud solutions, several technologies are becoming critical to manage and optimize these systems.
-
Kubernetes and Docker: These tools help in containerizing AI applications, allowing them to run consistently across different environments within the cloud, thereby improving deployment efficiency and simplifying scaling.
-
AutoML: Automation in creating, validating, and deploying models facilitates the optimization of AI workflows on cloud platforms.
-
Serverless Computing: This allows developers to build and run applications without managing the underlying infrastructure, focusing instead on application performance and innovation.
-
Edge AI: With computing done closer to the data source, edge AI solutions bridge the gap between cloud and local processing, enhancing response times and reducing bandwidth usage.
Challenges of Transitioning to Cloud-Based AI
Despite the benefits, there are challenges to consider when deploying AI systems to the cloud.
-
Data Privacy and Compliance: Moving data to the cloud requires stringent compliance with data protection regulations like GDPR.
-
Latency Issues: For applications requiring instant processing, cloud solutions might introduce latency that affects performance. This is where hybrid approaches utilizing both cloud and edge computing prove valuable.
-
Vendor Lock-In: Relying on a single cloud provider can limit flexibility and negotiating power, emphasizing the need for multi-cloud strategies.
-
Skill Gap: There is a growing demand for expertise in cloud technologies, creating a bottleneck in skills necessary for efficient migration and management of cloud-based AI systems.
The Future Outlook
The future of AI, facilitated by cloud computing, looks promising with several trends emerging to further enhance capabilities and adoption.
-
Federated Learning: This approach involves training AI models across decentralized devices without data sharing, ensuring privacy while improving model performance by leveraging cloud coordination.
-
Quantum Computing Integration: As quantum systems become more practical, their integration with cloud frameworks may redefine computational capabilities, tackling previously unsolvable problems.
-
AI as a Service (AIaaS): Offering AI tools and models as services in the cloud will enable more organizations to integrate smart capabilities into their operations seamlessly.
As businesses and technologies evolve, the synergy between AI and cloud computing will continue to be a focal point for innovation, enabling smarter and more efficient solutions in the realm of distributed AI systems.