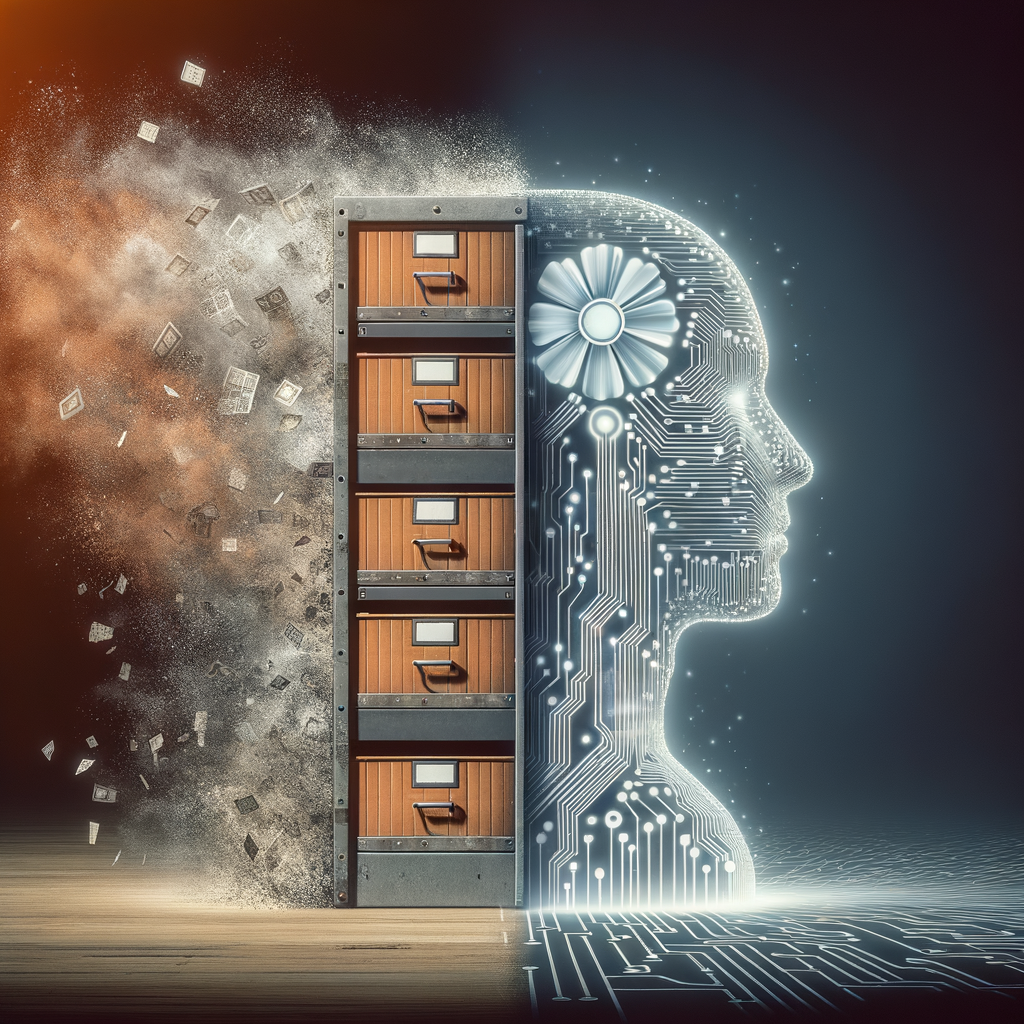
From Archival Dust to Digital Future: Reimagining Data Engineering with AI
Explore the transformative role of AI in the field of data engineering, from managing legacy data systems to creating agile, future-ready data infrastructures. Delve into the techniques that are revolutionizing data processes and discover how AI tools are enhancing data quality and insights.
From Archival Dust to Digital Future: Reimagining Data Engineering with AI
In the ever-evolving landscape of technology, data engineering has emerged as a critical field that supports the infrastructure and process of handling large-scale data collections. Traditionally, data engineering has been a cumbersome task involving the handling of legacy data systems, ensuring clean data transformations, and building the right data pipelines essential for analytics and business intelligence. With Artificial Intelligence (AI) entering the data domain, the scene is set for a remarkable evolution.
What is Data Engineering?
Data engineering involves preparing data for analytical or operational uses. This includes designing, building, and managing the information or 'big data' infrastructure. These data sets are then processed and made accessible for analysis, including tasks which range from batch processing and ETL (Extract, Transform, Load) operations to real-time streaming and data warehousing.
The Current State of Data Engineering
Historically, data engineering required substantial manual operations. As organizations depended heavily on data to drive business decisions, data engineers spent much time pinpointing errors in data entry, standardizing inputs, managing ETL pipelines, and dealing with issues of scale and latency.
How AI is Pioneering a New Path
Today, AI is poised to redefine conventions in data engineering. Let's explore some critical areas where AI demonstrates significant impact:
1. Automating ETL and Data Transformation
AI-powered tools can now automate complex data transformation rules that were previously written by hand. These AI systems can learn from existing data transformations, suggest optimizations, and even execute operations to transform data into a 'ready-to-consume' form.
2. Data Quality and Governance
Ensuring data quality is integral to reliable decision-making. AI assists in cleansing data by identifying errors, locating outliers, and recommending corrections, thus maintaining high data integrity levels. Moreover, AI-driven approaches can help in data governance, ensuring compliance and giving data lineage a clarity that was traditionally hard to achieve.
3. Real-Time Data Processing
Organizations focus increasingly on real-time analytics, needing immediacy in their data processes. AI accelerates the adaption of stream technologies capable of handling and processing real-time data efficiently without traditional latency issues.
4. Enhancing Data Pipelines with Predictive Analytics
AI introduces the ability to predict failures and suggest adjustments in data pipelines before they cause issues. AI’s predictive capabilities help anticipate downtimes, optimize resource allocation, and ensure consistent data flow.
Challenges and Considerations
While the infusion of AI into data engineering promises abundant possibilities, organizations must also address challenges such as:
- Data Privacy: Ensuring that AI systems conform to data privacy laws and regulations as they handle sensitive information.
- Skill Gap: Bridging the skills gap in workforce with effective training programs to combine AI capabilities with existing data engineering knowledge.
- Cost Management: Weighing the cost-versus-benefit ratio of investing in AI technologies for data processes.
Conclusion
From archival data stored deep within legacy systems to agile data infrastructures that enable the modern digital economy, AI is a catalyst for change within data engineering. It elevates efficiency, accuracy, and scale, preparing the domain for future challenges. As more AI-driven data tools surface, we can expect data engineering to transcend into a more visionary, impactful era across industries.
By leveraging AI in data engineering, businesses can not only improve their current processes but also set a solid foundation for innovations. This imposes an essential path forward, one that transforms archival dust into a glittering asset—a veritable gold mine of insights waiting to be unearthed.