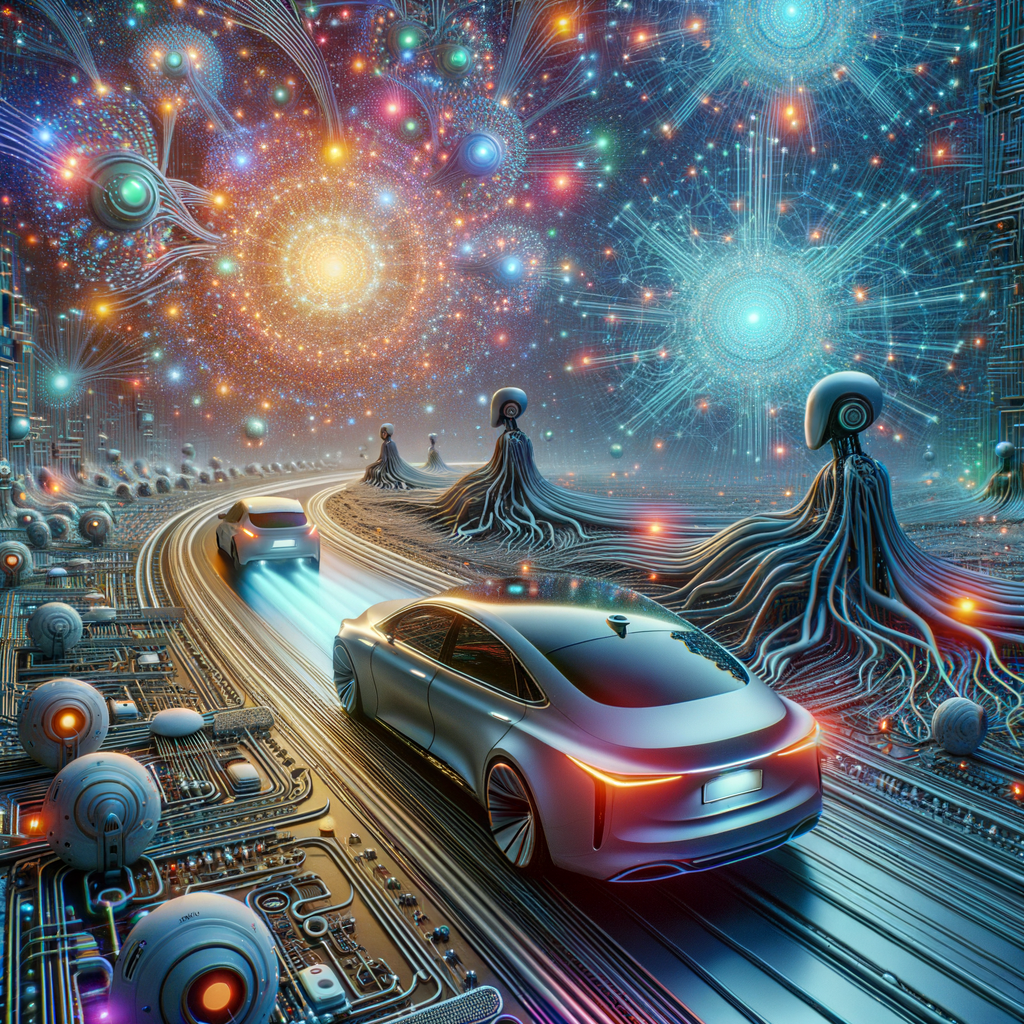
Exploring the Future of Deep Learning in Autonomous Vehicles
Dive into the intriguing world of autonomous vehicles and discover how deep learning is reshaping the landscape of transportation. This blog explores the latest advancements in AI and ML technologies that are driving innovation in self-driving cars. From navigation algorithms to real-time data processing, learn how these technologies are making autonomous vehicles a reality.
Exploring the Future of Deep Learning in Autonomous Vehicles
Introduction
Autonomous vehicles (AVs) -- self-driving cars, drones, and other pilotless crafts -- are no longer just a figment of science fiction. With rapid advancements in AI and machine learning, they are becoming an integral part of modern life. This article explores how deep learning is instrumental in this transformation.
The Role of Deep Learning
At the core of AV technologies is deep learning – a subset of artificial intelligence that mimics the way humans gain certain types of knowledge. Its applications in autonomous vehicles are numerous, from image recognition using cameras to interpreting radar and sensor data.
Image Recognition
Deep learning algorithms for image recognition enable vehicles to identify and interpret various objects on the road, such as other cars, pedestrians, traffic signals, and obstacles. This process is often carried out using convolutional neural networks (CNNs), which are exceptionally good at parsing visual data.
Sensor Data Analysis
Autonomous vehicles rely heavily on a fusion of data from cameras, radars, lidar, and ultrasonic sensors. Deep learning allows for the integration of this data into a coherent picture of the vehicle's surroundings, enabling accurate navigation and decision-making.
Algorithms Behind the Scenes
Navigation and Control
Autonomous vehicles employ complex deep learning-based algorithms for navigation. These include path planning, and making real-time decisions about acceleration, braking, and turning.
Real-Time Processing
The ability to process vast amounts of data in real-time is crucial for the safety and efficiency of AVs. Deep learning models are continuously trained and optimized to enhance their prediction and decision-making capabilities.
Challenges and Solutions
While the potential of deep learning in autonomous vehicles is vast, there are challenges that need addressing, including:
- Handling edges cases: Learning from uncommon road scenarios remains a challenge for AVs. Continuous learning and simulation training are crucial to cover these gaps.
- Regulatory Hurdles: AI models must adhere to safety standards and regulations, which often lag behind technological advancements.
- Data Privacy: As vehicles collect data about passengers and surroundings, privacy concerns must be resolved through secure data handling practices.
The Road Ahead
The future of autonomous vehicles powered by deep learning looks promising. With continuous research and innovation, the technology promises safer, more efficient transportation systems.
Conclusion
Deep learning is at the forefront of driving the future of mobility. As we advance towards fully autonomous systems, the symbiosis of AI and machine learning will be crucial in overcoming the complexities of real-world driving.