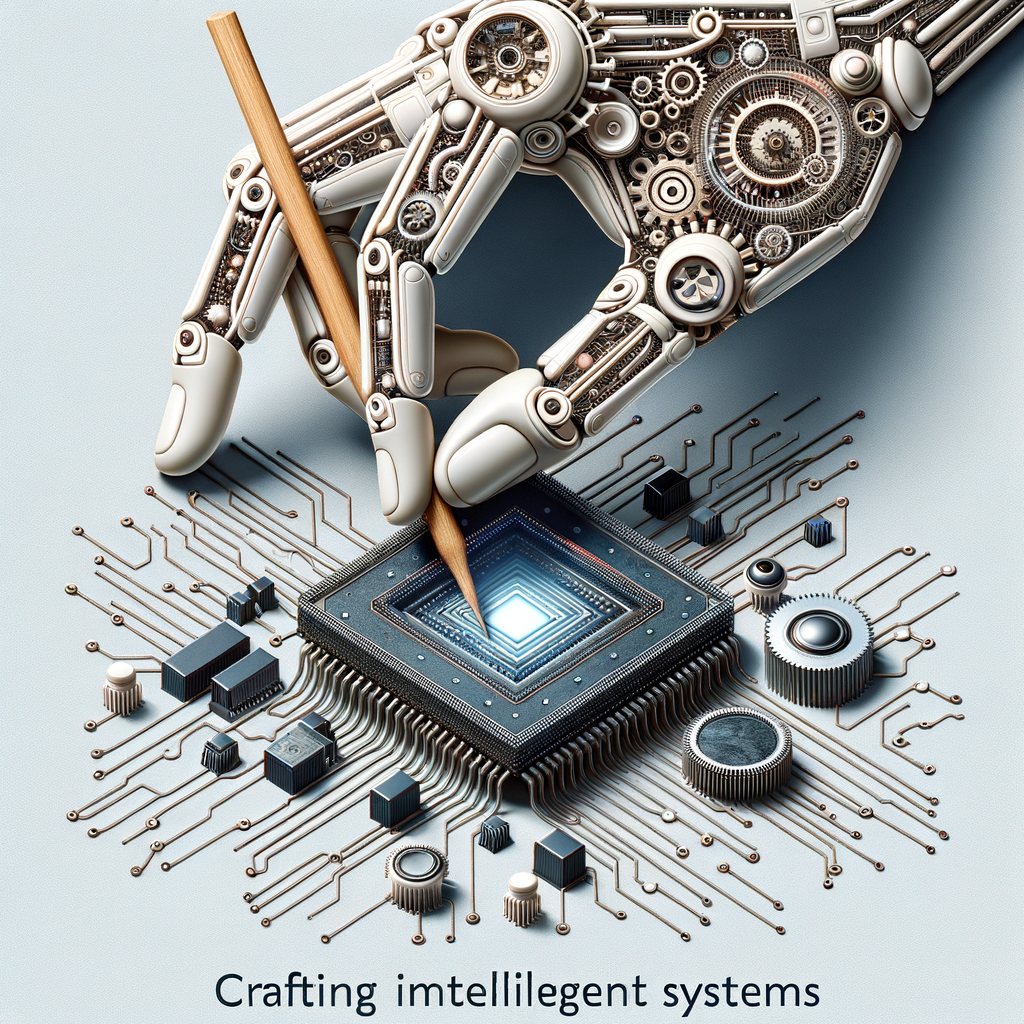
Crafting Intelligent Systems: A Deep Dive into Autonomous AI Infrastructure
Explore the intricacies of autonomous AI infrastructure, detailing the architecture, design principles, and practical applications that drive today's intelligent systems. Discover how these systems are transforming various industries and paving the way for a future powered by autonomous AI.
Crafting Intelligent Systems: A Deep Dive into Autonomous AI Infrastructure
In recent years, the landscape of artificial intelligence has seen a remarkable evolution with the advancement of autonomous systems. Autonomous AI infrastructure stands at the forefront of this transformation, enabling the creation of intelligent systems capable of operating independently, making decisions, and performing tasks without human intervention. In this deep dive, we will explore the different components that constitute autonomous AI infrastructure, understand its applications across various industries, and delve into future advancements that may redefine its role in the technology ecosystem.
Understanding Autonomous AI Infrastructure
At its core, autonomous AI infrastructure comprises a network of interconnected systems that support the execution and adaptation of AI-driven tasks. This infrastructure includes computational power, storage capabilities, robust networking, and sophisticated algorithms. The integration of these elements allows for seamless data flow, real-time processing, and reduces latency, facilitating continuous learning and evolution of AI models.
Key Components
-
Computational Hardware: At the heart of any AI system is its computational capability. Autonomous systems rely on a mix of CPUs, GPUs, TPUs, and specialized hardware to process complex algorithms efficiently.
-
Network Infrastructure: High-speed connectivity is crucial for data transfer between nodes in an AI system. Advanced network architectures ensure low latency, high bandwidth, and reliable communication channels, essential for real-time decision-making.
-
Data Storage Solutions: The data fuelling AI models is vast, varied, and ever-growing. Distributed storage mechanisms, such as cloud storage and edge computing, allow for scalable and efficient data management.
-
AI Models and Algorithms: Driving the intelligence in these systems are the neural networks and learning algorithms that process data, recognize patterns, and make informed decisions. These models leverage techniques from machine learning, deep learning, and reinforcement learning to achieve autonomy.
-
Security and Compliance Frameworks: As these systems grow in complexity, ensuring security and adherence to compliance standards becomes critical. Advanced encryption, anomaly detection, and continuous monitoring are integral to safeguarding AI infrastructures.
Practical Applications
Transportation
One of the most visible applications of autonomous AI infrastructure is in self-driving vehicles. These systems integrate AI with sensor technologies, such as LIDAR and computer vision, to navigate roads, interpret traffic conditions, and ensure passenger safety.
Healthcare
In the healthcare industry, AI infrastructure empowers diagnostic systems to analyze medical images, predict patient outcomes, and recommend personalized treatment plans autonomously, improving patient care efficiency.
Industrial Automation
Factories and production units are increasingly leveraging autonomous AI systems to optimize process flows, manage supply chains, and maintain equipment proactively through predictive maintenance.
Financial Services
In finance, autonomous AI infrastructure is being employed to develop smart advisory systems, fraud detection mechanisms, and algorithmic trading strategies, providing a competitive edge and enhancing decision-making.
Future Directions
As technology progresses, the future of autonomous AI infrastructure is poised for significant advancements. The integration of quantum computing promises to further accelerate computational capabilities, while advancements in AI ethics and explainability will improve trust and transparency in these systems.
Additionally, the rise of 5G and beyond will open new avenues for the deployment of AI systems in real-time, distributed scenarios, such as smart cities and IoT ecosystems.
Conclusion
Autonomous AI infrastructure represents a crucial pillar in the ongoing AI revolution. By understanding its components, applications, and potential future developments, we can better prepare for a future where intelligent systems play an integral role in everyday life. Adopting and adapting these technologies within ethical frameworks and societal norms will be essential for their successful integration and benefit to humanity.