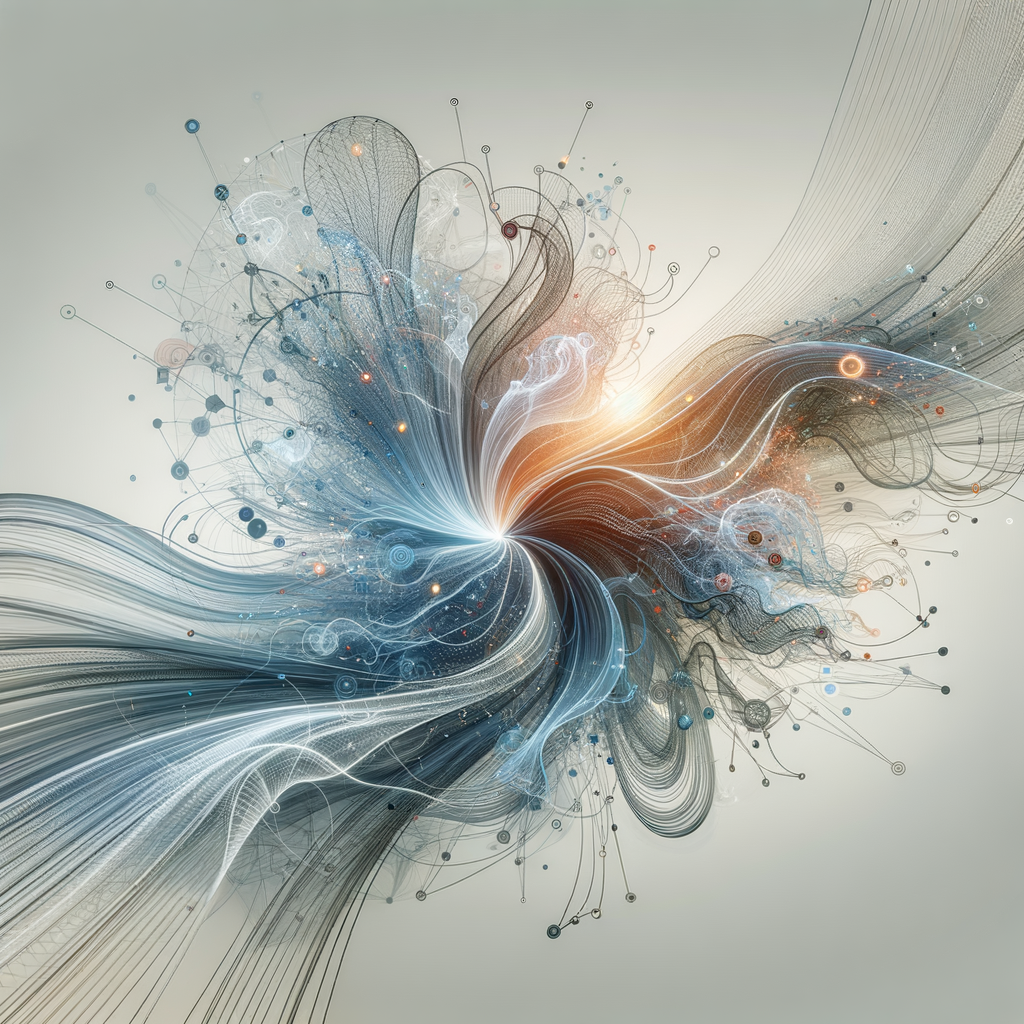
Beyond Traditional Text Generation: The Future of Multi-Modal AI Systems
Dive into the world of multi-modal AI systems that are revolutionizing how machines understand and interact with various forms of data beyond text, enabling smarter decision-making and enhanced user experiences across diverse industries.
Beyond Traditional Text Generation: The Future of Multi-Modal AI Systems
Introduction
As the artificial intelligence landscape expands, we witness the evolution of systems that go beyond traditional text-based data processing to incorporate a wider range of data types. Multi-modal AI systems promise to revolutionize not only how machines understand human communication but also how they interact with and comprehend diverse datasets that include images, audio, video, and sensor data. This integration of multiple data types allows AI to engage in more complex and meaningful interactions, making it invaluable across various industries.
Understanding Multi-Modal AI
Multi-modal AI refers to the capability of artificial intelligence systems to process and interpret input from multiple data modalities—such as text, visuals, sound, and other signal forms—simultaneously. Traditional AI systems, particularly those for natural language processing (NLP), were often limited to text, which restrained their potential. With the advent of technologies that can process and integrate data from multiple sources, AI can offer richer insights and enable more sophisticated user interactions.
Key Components of Multi-Modal AI Systems
- Data Fusion: The integration of various data types into a coherent representation, allowing the extraction of meaningful insights that would be difficult to attain through isolated modalities.
- Deep Learning: Algorithms such as convolutional neural networks (CNNs) and recurrent networks that play a crucial role in processing different data types. They help in extracting features from images, recognizing speech patterns, and understanding complex textual content.
- Natural Language Understanding: Enhancing current NLP capabilities to contextualize text with multimedia elements for superior understanding and interaction.
Applications Across Industries
-
Healthcare: Multi-modal AI can analyze medical texts, scans, and genetic data to assist in diagnostics and personalized treatment plans, thereby improving patient outcomes. It can integrate voice recognition for patient monitoring and automate the interpretation of imaging data with extraordinary precision.
-
Media and Entertainment: Enhancing user experiences through personalized content delivery, sentiment analysis of videos, and automatic labelling of visual content. AI can predict viewer preferences by analyzing viewing habits alongside textual content from user reviews.
-
Retail and E-commerce: Creating more engaging customer experiences by analyzing purchase histories, user feedback, and real-time interaction data to deliver personalized product recommendations. Computer vision technologies can assist in inventory management by identifying and categorizing products from images.
-
Autonomous Vehicles: Integrating data from cameras, LIDAR, radar, and GPS to understand the vehicular environment, make real-time driving decisions, and enhance safety features.
-
Education: Developing intelligent tutoring systems that provide customized learning experiences by analyzing textual content, student engagement via video analysis, and comprehension through auditory assessments.
Challenges in Multi-Modal AI Systems
Despite their promising potential, multi-modal AI systems face several technical and ethical challenges:
- Complexity of Integration: Effectively combining different modalities requires sophisticated algorithms and high computing resources.
- Quality of Data: Ensuring that data from all modalities are accurate and relevant to maintain the integrity of AI predictions and decisions.
- Privacy and Security: Managing large volumes of personal and sensitive data demands stringent privacy measures and robust security frameworks.
The Future Landscape
As AI continues to evolve, multi-modal systems will become more prevalent and sophisticated. Advances in sensor technology, computational power, and deep learning will drive these systems to new heights. We can expect enhanced natural interaction with AI, leading to systems that not only process human language proficiently but also ‘see’, ‘hear’, and ‘understand’ the world in much the same way humans do.
Multi-modal AI heralds an era of unprecedented capabilities across sectors. By embracing and overcoming current challenges, industries can leverage AI to uncover deeper insights, foster better understanding, and cultivate more intuitive user interactions.
Conclusion
Multi-modal AI systems represent the next frontier in the artificial intelligence journey. Their ability to process and synthesize information from multiple data types will transform numerous fields, from healthcare and entertainment to education and retail. As these systems grow more advanced, the enhanced capabilities will lead to innovations that could redefine how we interact with technology and, by extension, each other.
In future explorations, researchers and developers must address the challenges of data quality, integration complexity, and ethical considerations to ensure these powerful systems benefit society as a whole, paving the way for AI solutions that are both intelligent and profoundly human.