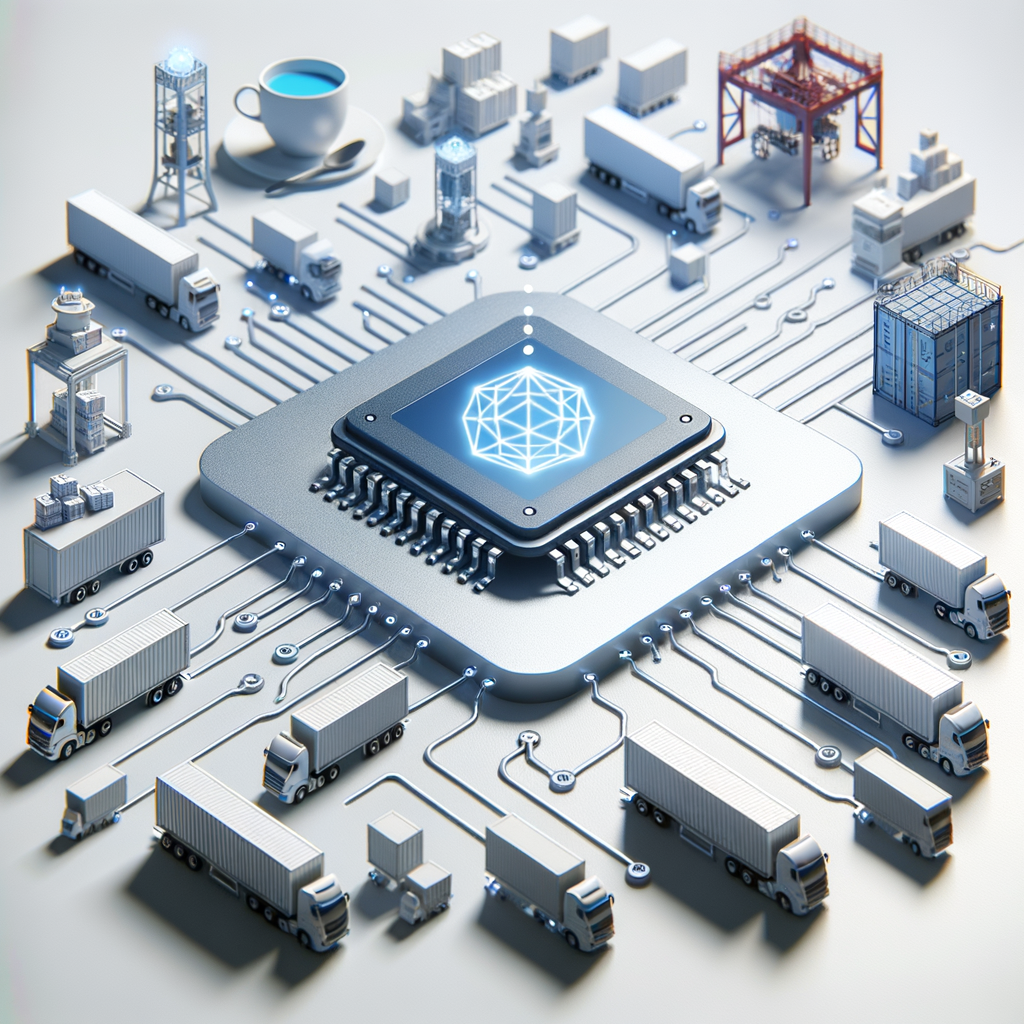
Beyond Traditional Algorithms: How AI is Reinventing Supply Chain Management
Explore the transformative power of AI in supply chain management, revolutionizing efficiency and decision-making processes. Discover how machine learning models are optimizing logistics, inventory management, and demand forecasting to create agile, resilient supply chains for the future.
Beyond Traditional Algorithms: How AI is Reinventing Supply Chain Management
In today's globalized world, supply chain management has become increasingly complex, demanding robust solutions that can keep up with rapidly changing markets. Artificial Intelligence (AI) is at the forefront of this revolution, providing innovative strategies to streamline operations and enhance decision-making agility. In this blog post, we'll explore how AI technologies, particularly machine learning and data analytics, are reshaping supply chain management.
The Evolution of Supply Chain Management
Traditional Vs. AI-Powered Approaches
Traditionally, supply chains have been managed through a combination of manual oversight and legacy systems that often fail to adapt quickly to market changes. AI introduces a new paradigm, where machine learning algorithms can predict demand trends, optimize logistics routes, and minimize operational bottlenecks.
Key Drivers Behind AI Adoption
The push towards AI-driven supply chain management stems from the need to enhance visibility, improve accuracy in demand planning, and create more resilient systems capable of adapting to unexpected disruptions. These technologies enable companies to make data-driven decisions with unprecedented speed and precision.
Machine Learning in Demand Forecasting
One of the most critical aspects of supply chain management is demand forecasting. AI leverages vast datasets to predict future demand with greater accuracy. Machine learning models analyze historical sales data, market trends, and external factors such as economic indicators and weather patterns to refine forecasts continuously.
Case Studies and Applications
Several industry leaders have successfully integrated AI-powered forecasting into their supply chains. For instance, retail giants like Walmart and Amazon utilize predictive analytics to dynamically adjust their inventory, ensuring they meet customer demand without overstocking.
Optimizing Inventory Management
AI supports real-time inventory monitoring, helping businesses maintain optimal stock levels. Advanced inventory management systems use AI to automate reordering processes and identify inefficiencies in storage and distribution.
Enhancements through AI
By implementing AI, companies can lower holding costs, reduce waste through better input-output management, and improve order fulfillment accuracy. Predictive maintenance, powered by AI, also plays a pivotal role in ensuring equipment and machinery are operating efficiently, reducing downtime and maintenance costs.
Logistics and Route Optimization
Logistics is another area where AI's impact is profound. AI systems can analyze traffic data, weather conditions, and fleet performance metrics to optimize delivery routes, save fuel costs, and enhance delivery times.
Real-World Implementations
Advanced logistics systems, such as those implemented by UPS, utilize AI for route optimization, enabling the company to deliver packages faster and reduce its carbon footprint.
Building Resilient Supply Chains
In an era characterized by uncertainties—from pandemics to geopolitical tensions—resiliency in supply chain management is of paramount importance. AI supports the development of systems that anticipate disruptions and provide adequate contingency planning.
Collaborative Networks and AI
AI facilitates the creation of collaborative networks, allowing businesses to connect with suppliers more efficiently and share information seamlessly. This connectivity enhances transparency and collaboration across the entire supply chain.
The Challenges of AI Integration
Despite its advantages, integrating AI into supply chains presents challenges, including data privacy concerns, the need for high-quality data, and the complexity of implementing AI solutions into existing infrastructures.
Overcoming Integration Barriers
Businesses need to invest in robust data management frameworks and foster a culture of innovation to harness AI's full potential. Partnering with technology providers and investing in employee training are crucial steps towards successful AI adoption.
The Future Landscape of AI in Supply Chain Management
As technology advances, AI in supply chain management is likely to focus on sustainability and ethical practices, using AI for efficient resource utilization and minimizing the environmental impact of business operations.
Innovations on the Horizon
Future advancements may include the integration of blockchain for enhanced tracking and AI-driven autonomous vehicles for deliveries. These innovations promise to further streamline the supply chain processes, creating seamless, efficient, and agile supply chains.
Conclusion
AI is poised to redefine supply chain management, providing dynamic solutions that enhance efficiency, agility, and resiliency. As companies become more adept at integrating these technologies, we can anticipate a future where supply chains are not only optimized for performance but are also sustainable and adaptable to the challenges of a rapidly changing world.