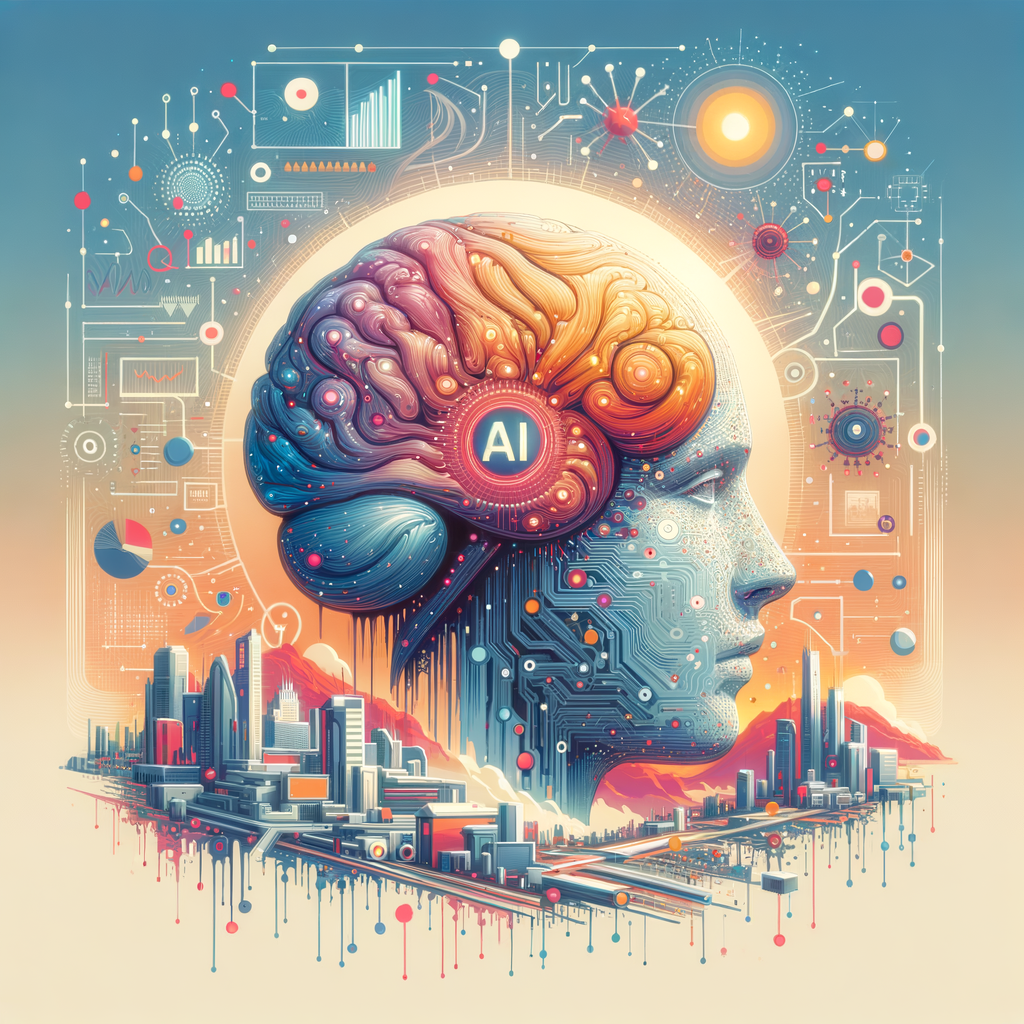
Beyond Traditional AI: Exploring Neuro-Symbolic Systems
Delve into the fascinating world of neuro-symbolic systems, where the integration of symbolic reasoning and neural networks promises to redefine AI capabilities. This blog post explores the synergy between these two paradigms, highlighting their potential to enhance decision-making processes, improve problem-solving, and foster explainability in AI applications.
Beyond Traditional AI: Exploring Neuro-Symbolic Systems
Artificial intelligence is advancing at an unprecedented pace, and with it, novel architectures and methodologies are emerging to tackle the limitations of current AI systems. One such promising approach is neuro-symbolic systems, which merge the strengths of symbolic reasoning with the powerful learning capabilities of neural networks. This fusion promises to address some of the pressing challenges in AI, such as explainability, interpretability, and efficient learning from minimal data.
Understanding the Core Concepts
Symbolic AI, often characterized by rule-based systems, excels in logical reasoning tasks where transparency and explainability are crucial. Unlike neural networks, which learn patterns from vast amounts of data and often operate as black boxes, symbolic AI provides clear insights into decision-making processes. Conversely, neural networks shine in processing unstructured data such as images and natural language, drawing on their ability to learn complex patterns and representations.
Neuro-symbolic systems aim to leverage the best of both worlds. By integrating symbolic reasoning into the learning pipeline of neural networks, it becomes possible to build hybrid models that bring together the interpretability of symbol-based systems with the adaptability and scalability of connectionist models.
Key Advantages of Neuro-Symbolic Systems
The integration of neuro-symbolic approaches brings several notable advantages:
-
Enhanced Explainability: By incorporating symbolic logic into neural architectures, these systems provide reasoning paths that are easier to interpret and debug.
-
Improved Generalization: Symbolic knowledge can be utilized to guide neural networks, offering a form of inductive bias that improves learning efficiency and helps models generalize from fewer examples.
-
Robustness and Flexibility: Neuro-symbolic systems demonstrate robustness in dynamic environments by maintaining a blend of structured and flexible problem-solving techniques.
Real-World Applications and Case Studies
The convergence of neural and symbolic systems is already finding applications across diverse domains:
-
Healthcare: Diagnostic systems powered by neuro-symbolic AI combine clinical guidelines (symbolic reasoning) with patient data (neural networks) to provide accurate and explainable medical predictions.
-
Automated Planning: Knowledge-intensive domains like robotics and logistics benefit from the planning capabilities of symbolic AI, which, when enhanced by neural learning, lead to more efficient pathfinding and task execution.
-
Natural Language Processing (NLP): Understanding contextual and logical relationships in language can be significantly improved by blending symbolic semantics with neural network capabilities.
Challenges and Future Directions
Despite their potential, neuro-symbolic systems face certain challenges. Ensuring seamless integration, handling inconsistencies between symbolic rules and learned patterns, and maintaining computational efficiency are ongoing areas of research.
The future of neuro-symbolic AI is promising, with more research focused on creating adaptive algorithms that can dynamically switch between symbolic reasoning and neural processing. As these systems continue to evolve, they hold the key to unlocking more efficient, understandable, and reliable AI solutions.
Conclusion
Neuro-symbolic systems mark a transformative step in the evolution of artificial intelligence, promising to bridge the gap between understanding and learning in a way that previous architectures could not. By translating complex, large-scale data into comprehensible, actionable insights, they have the potential to revolutionize how AI interacts with the world.
At the heart of this transformation is the quest for building AI that not only mirrors human intelligence but collaborates with it more seamlessly. As research in neuro-symbolic AI progresses, we are likely to see profound changes in how machines aid human decision-making and creativity.