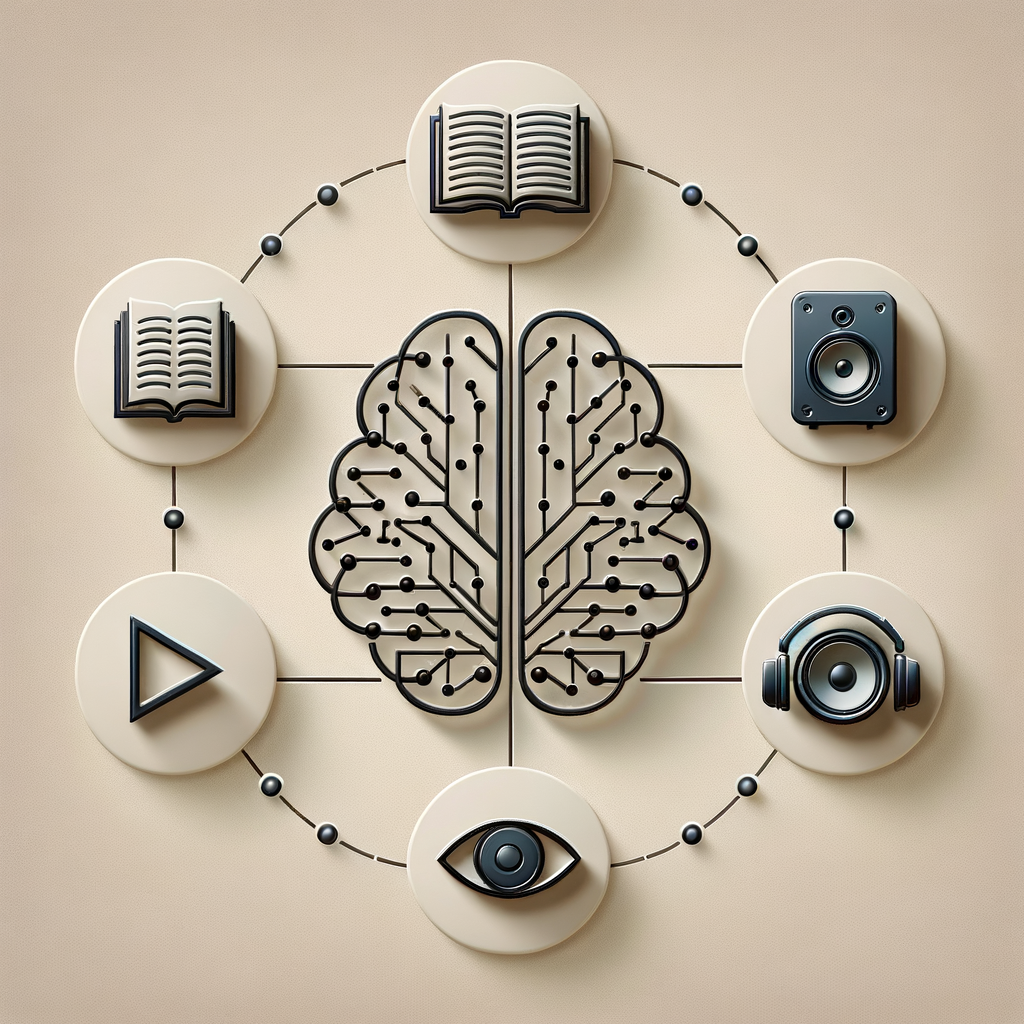
Beyond Text: Revolutionizing AI with Multimodal Learning
This blog delves into the fascinating world of multimodal learning, exploring how AI incorporates and synthesizes data from multiple sources like text, audio, and video to create more holistic, accurate, and contextually aware models. We'll look at the groundbreaking research and applications that are paving the way for a new era of intelligent systems.
Beyond Text: Revolutionizing AI with Multimodal Learning
Introduction
In the ever-evolving field of artificial intelligence, there exists a myriad of ways to ingest, interpret, and produce data. Traditionally, AI and machine learning models have focused on a single type of data at a time—be it text, image, audio, or video. However, recent advancements have led to the forefront of innovation in multimodal learning, a paradigm that seeks to integrate multiple forms of data into unified models. This approach not only mimics the human way of processing information but also paves the way for more robust, accurate, and versatile AI systems.
Understanding Multimodal Learning
At its core, multimodal learning is about combining data from multiple modalities. Imagine a virtual assistant that can not only understand your verbal instructions but also interpret your visual cues. This fusion allows for a richer understanding and interpretation of inputs, culminating in more accurate and contextually apt responses or actions.
Key Components
- Data Integration: Combining different types of data from various sources.
- Data Synchronization: Ensuring temporal alignment for datasets, which is crucial especially when dealing with audio-visual data.
- Cross-Modal Learning: Utilizing one form of data to enhance the understanding of another.
The Importance of Multimodal AI
The benefits of mastering multimodal learning extend across various applications:
- Healthcare: Multimodal systems can improve diagnostic accuracy by combining imaging data, patient health records, and even patient interviews.
- Autonomous Vehicles: Vehicles that synthesize data from visual sensors, radar, and even real-time traffic information can achieve significantly higher levels of reliability and safety.
- Smart Assistants: Voice-activated systems that can 'see' and 'hear' in tandem offer a rich interaction experience far beyond simple voice command execution.
Challenges in Multimodal Learning
- Data Alignment: Different forms of data often come with different sampling rates and temporal sequences.
- Model Complexity: Integrating multiple data sources increases the computational load and model complexity.
- Data Privacy and Security: Multimodal systems often require access to a breadth of personal data, raising significant privacy concerns.
Innovations and Research
Seminal research in this field includes developments in representational learning that bridge gaps between modalities, enabling machines to interpret complex scenes holistically. Innovations like Google's BERT, which integrates text with image data for richer NLP applications, are pushing boundaries further than ever expected.
Future Prospects
The horizon for multimodal AI is vast. As the technology matures, we anticipate breakthroughs in areas such as:
- Augmented Reality: Enhanced AR experiences by creating seamless integrations between real-world data and computer-generated modalities.
- Personalized Learning Systems: Education platforms that adapt to both auditory and visual inputs to customize educational content per individual learner's needs.
- Immersive Entertainment: Creation of highly interactive storytelling adventures where sensory inputs tailor endings.
Conclusion
As we combine and synchronize multiple data streams, we unlock the true potential of AI, transforming machines from simple task performers into insightful, interactive companions capable of understanding and responding to the rich tapestry of human experience. Multimodal learning not only enhances machine intelligence but represents a significant step towards creating AI systems that understand the world as deeply and richly as humans do.
Call to Action
What are your thoughts on the potential and implications of multimodal AI? How can we address the ethical and technical challenges presented? Share your insights in the comments below, and stay tuned for more on the innovations driving tomorrow's technology.