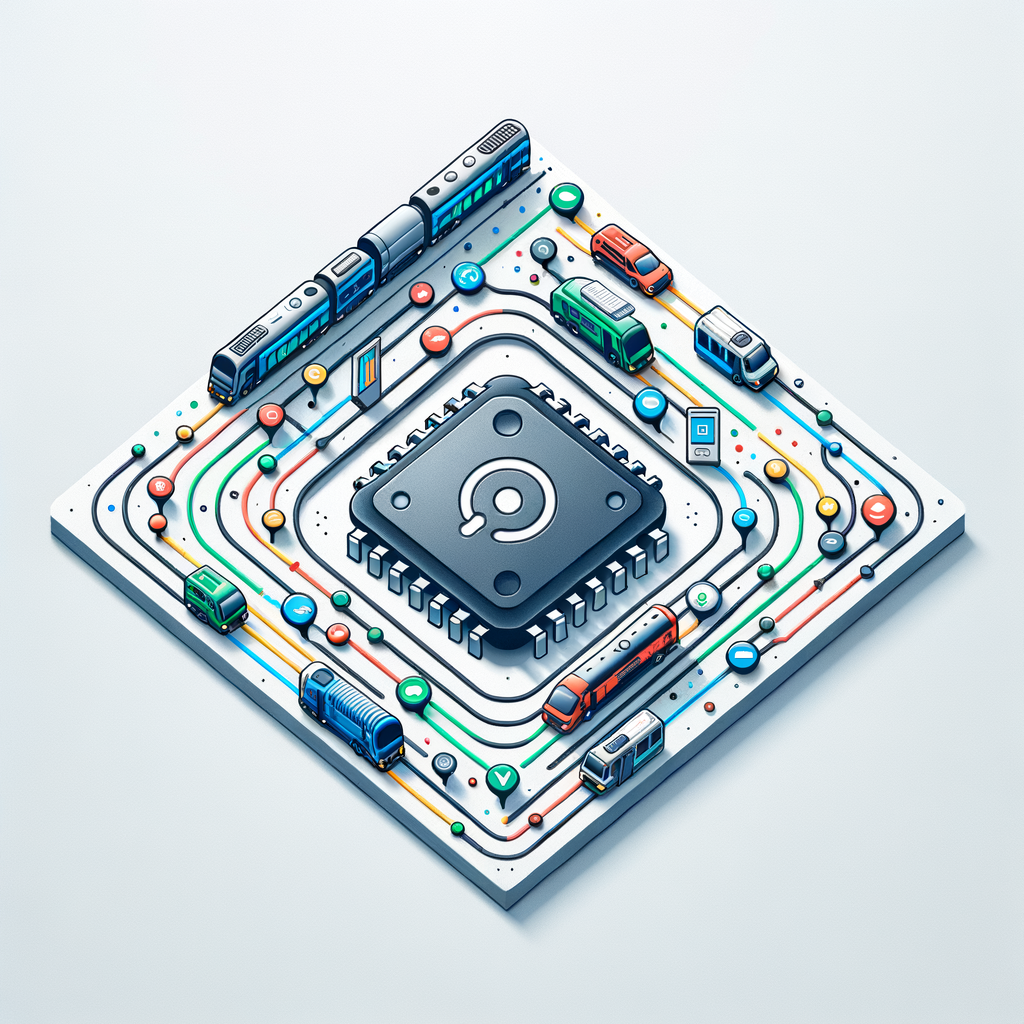
Beyond Converse: Breathing Life into AI Conversations with Multimodal Transit Systems
Explore the innovative realm of AI-powered multimodal transit systems where language, images, sound, and real-time analytics merge seamlessly. This blog dives into how these advanced systems are evolving to provide more interactive, intuitive, and efficient communication landscapes within AI ecosystems, potentially revolutionizing industries ranging from customer service to interactive storytelling and beyond.
Beyond Converse: Breathing Life into AI Conversations with Multimodal Transit Systems
In the age of AI, conversations are taking a new turn, shifting from purely text-based exchanges to dynamic, multimodal interactions that incorporate language, images, video, sound, and even real-time analytics. This transformation is driven by AI-powered multimodal transit systems, which are increasingly being adopted across various fields to enhance interaction accuracy, user experience, and contextual relevance. This article explores the evolution, components, and applications of these innovative systems in AI-driven interaction landscapes.
The Evolution of AI Conversations
Traditional AI conversations have primarily focused on text-based chatbots and virtual assistants, using natural language processing (NLP) to understand and generate human language. While effective, these systems often fall short in comprehending nuanced contexts or conveying rich information that visual or auditory cues can provide. As a result, there has been a growing shift toward multimodal AI systems, which aim to enrich conversations by integrating multiple types of data inputs and outputs.
Multimodal AI: A New Paradigm
Multimodal AI systems capture and interpret data from various sources—text, voice, images, and sensor data—to create a more holistic understanding of input information. By combining these diverse data types, AI can produce more informed, contextually aware responses, ultimately leading to more meaningful interactions.
Key Components of Multimodal Transit Systems
-
Data Fusion: The process of integrating diverse data inputs (e.g., text, audio, images) to form a comprehensive dataset that enhances understanding and decision-making.
-
Deep Learning Algorithms: Utilized to process and learn from multimodal data, these algorithms enable AI to recognize patterns, interpret nuances, and provide accurate predictions across different contexts.
-
Real-time Analytics: Vital for instantly processing and analyzing data inputs, these analytics allow AI to adapt and respond promptly, keeping interactions fluid and contextually relevant.
-
Interactive Interfaces: User interfaces that allow seamless engagement across different modalities, offering users options to interact using text, voice, or visual elements, according to their preferences.
Applications of Multimodal Transit Systems
1. Enhanced Customer Support
Businesses are adopting multimodal systems in customer service scenarios, using AI to handle complex queries more effectively by understanding customer inputs across different formats—whether they provide information through text, voice, or images.
2. Interactive Storytelling
In the media and entertainment industry, multimodal AI is enabling richer interactive storytelling experiences. Users can engage with content using various inputs, offering a more immersive experience by blending text, sound, and visual media.
3. Accessibility Innovations
Multimodal transit is increasingly being utilized to improve accessibility for individuals with disabilities. For instance, applications that combine voice recognition with visual cues can assist users in navigating digital environments more effectively regardless of limitations in one sensory modality.
The Future of AI Conversations
The future of AI conversations lies in further enhancing the synergy between different modalities, making interactions not only more effective but also more human-like. As advancements in AI continue to progress, these systems will not only support businesses in achieving better customer engagement but will also pave the way for broader societal impacts including education, healthcare, and social inclusion.
Challenges and Considerations
While promising, developing effective multimodal systems comes with challenges. Ensuring data privacy, managing computational demands, and overcoming cultural or linguistic biases require ongoing research and practical solutions.
Conclusion
As we march into the future, AI-powered multimodal transit systems hold immense potential to transform the way humans and machines interact. By merging diverse data types and crafting seamless interaction experiences, these systems promise a future where AI conversations are not just interactive, but truly engaging and comprehensive.
By continuing to push the envelope, embracing technological innovations, and addressing challenges head-on, we can leverage these cutting-edge systems to unlock new possibilities and forge a more connected, interactive world.