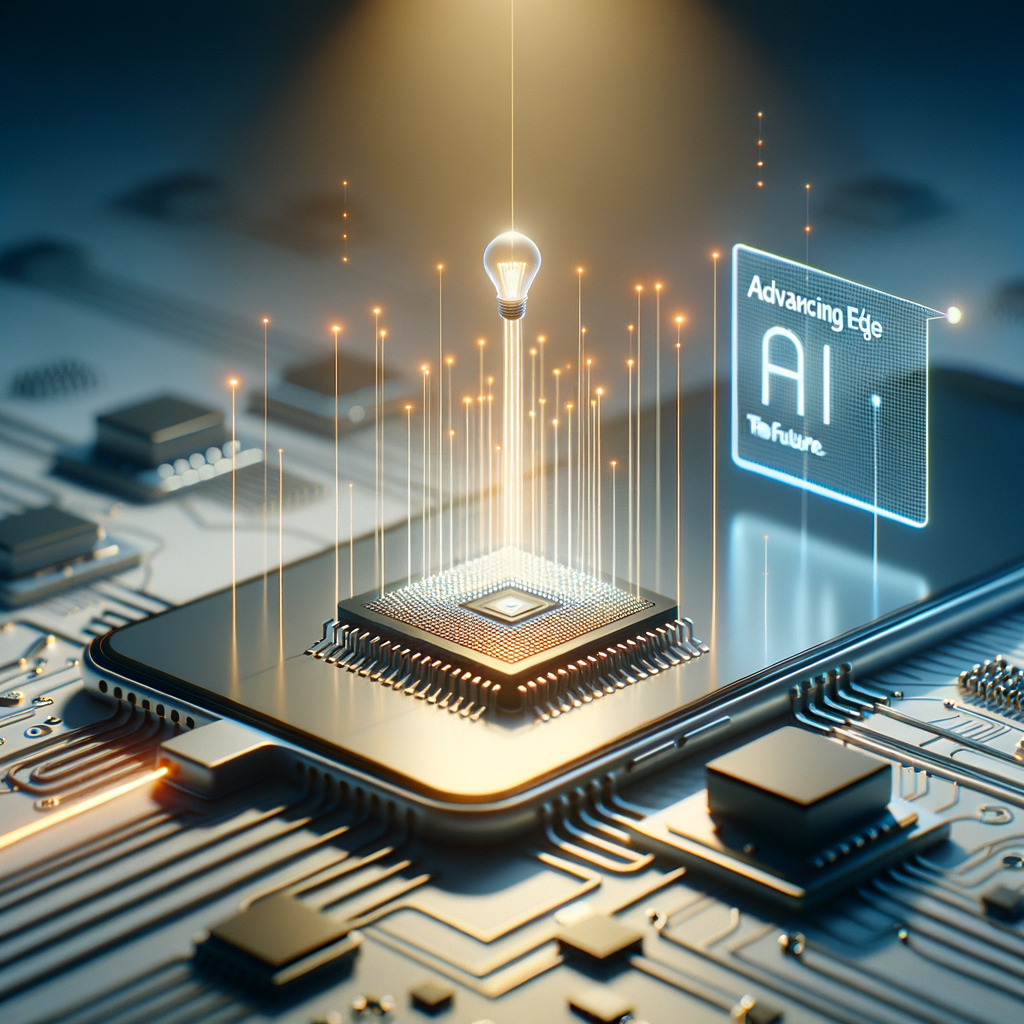
Advancing Edge AI: The Future of On-Device Intelligence
Explore the transformative impact of Edge AI technology as it moves artificial intelligence processing closer to data sources like smartphones and IoT devices. This blog covers the advantages of Edge AI, including low latency and enhanced privacy, and its applications across various industries such as healthcare, automotive, and smart devices. Learn how Edge AI is paving the way for more efficient, responsive, and secure tech ecosystems.
Introduction
In recent years, the development of artificial intelligence (AI) technologies has gained considerable momentum, mainly driven by advancements in cloud computing. However, the increasing demand for real-time data processing and enhanced privacy has set the stage for a new era in AI: Edge AI. This technology aims to bring computational capabilities closer to where data is generated, providing numerous benefits such as reduced latency, improved privacy, and decreased dependency on centralized cloud services. This blog post deep dives into the evolution and benefits of Edge AI, its applications across various fields, and the challenges faced in its deployment.
The Evolution of Edge AI
Traditionally, AI processing has been largely centralized with reliance on powerful cloud servers. This model works well for many applications but introduces limitations like high latency, increased bandwidth usage, and privacy concerns. Edge AI offers a solution by processing data on local devices, such as smartphones, IoT devices, and embedded systems. This shift towards on-device intelligence is made possible by the growing capability of hardware accelerators like GPUs and TPUs, improved deep learning algorithms, and optimization of AI models for low-power environments.
Advantages of Edge AI
1. Low Latency:
Edge AI eliminates the need for data to be sent to a remote server for processing, significantly reducing the response time for AI-driven applications. This is particularly advantageous for time-sensitive applications such as autonomous vehicles and real-time health monitoring systems.
2. Enhanced Privacy:
By keeping data local to the device, Edge AI helps protect user privacy. Sensitive information does not need to leave the device, lowering the risk of breaches and unauthorized access.
3. Bandwidth Efficiency:
With less data transmitted to cloud servers, Edge AI reduces bandwidth usage and associated costs. This efficiency is critical for the massive datasets generated by IoT devices.
4. Reliability:
Edge AI systems can operate independently of a stable internet connection, making them more reliable in environments with poor connectivity. This capability is valuable for applications in remote locations or critical infrastructure.
Applications of Edge AI
Edge AI finds applications in a wide range of industries:
1. Healthcare:
Wearable devices utilizing Edge AI can monitor vital signs in real-time, providing immediate feedback and reducing the need for continuous internet connectivity. This on-device processing can lead to faster diagnosis and more personalized healthcare solutions.
2. Automotive:
In autonomous vehicles, Edge AI is crucial for executing real-time object recognition and decision-making processes. This localized processing ensures faster response times, which is vital for safety and efficiency.
3. Smart Devices:
Smart home devices like voice assistants and cameras benefit from Edge AI through faster processing of user commands and improved data security by not uploading sensitive audio and video feeds to the cloud.
4. Industrial IoT:
Manufacturing processes can utilize Edge AI to perform predictive maintenance by analyzing sensor data on-site, leading to reduced downtime and enhanced productivity.
Challenges and Considerations
Implementing Edge AI presents several challenges:
1. Hardware Constraints:
Edge devices often have limited power and compute capabilities, requiring highly optimized AI models to run efficiently.
2. Data Management:
Handling data across many edge devices necessitates robust data management strategies to ensure consistency and accuracy in model predictions.
3. Security:
While Edge AI enhances privacy, ensuring secure model updates and protecting against potential attacks on edge devices remain critical concerns.
4. Integration with Cloud:
Seamless integration between edge and cloud computing resources is needed for applications that require both local processing and additional cloud resources.
Conclusion
Edge AI represents a paradigm shift in how we process and interact with data, moving away from centralized systems to more distributed, efficient, and privacy-conscious models. As hardware capabilities continue to improve and AI models become more optimized for edge deployments, we can expect to see an increasing number of applications harnessing the power of Edge AI. This technological shift promises to unlock new possibilities across various sectors, redefining how we experience and leverage artificial intelligence in our daily lives.
The journey of Edge AI has just begun, and its potential to reshape the landscape of AI-driven innovations is immense. As the technology evolves, it will create exciting new opportunities while addressing contemporary challenges in data processing, security, and scalability.