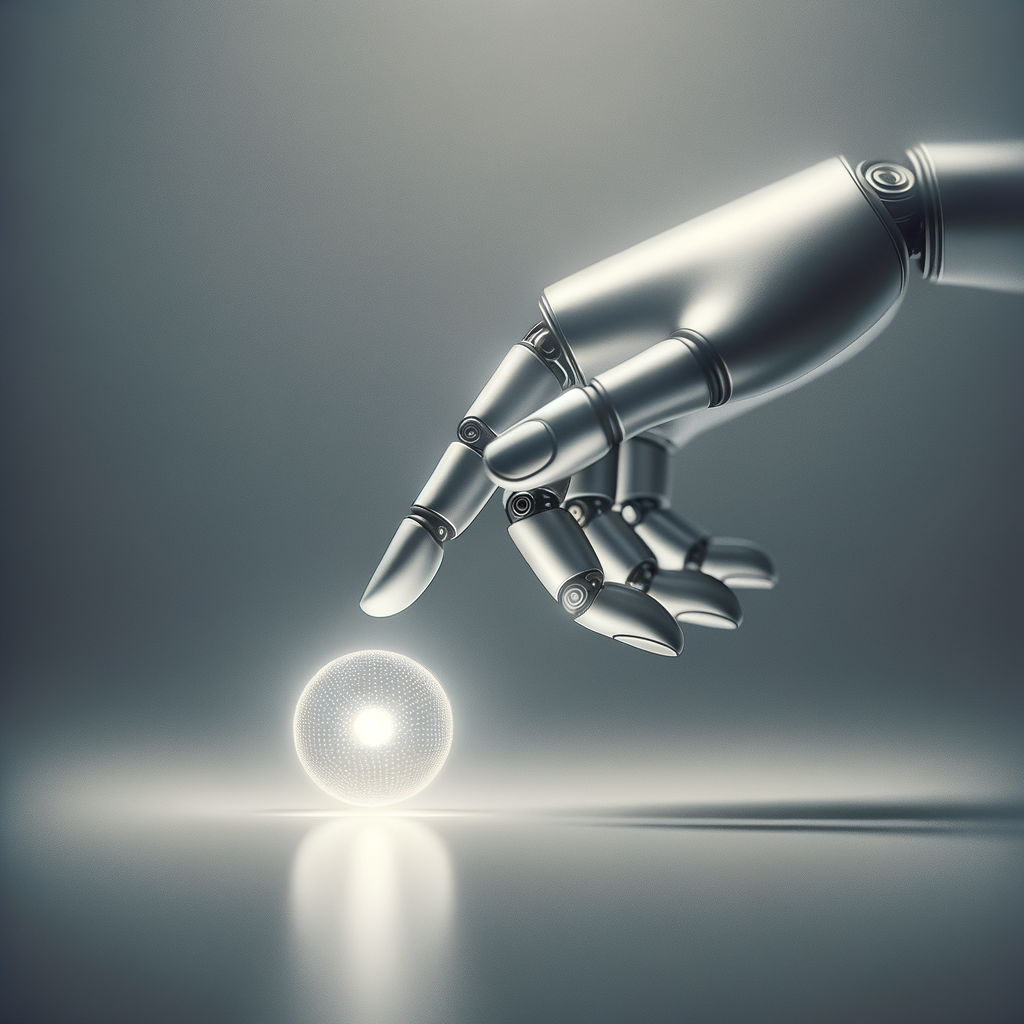
Accelerating AI Innovation: The Role of Auto-Generated Data in Machine Learning
Discover how auto-generated data is reshaping the landscape of machine learning and AI development. This blog explores the techniques and technologies behind auto-generating datasets, including its impact on efficiency and accuracy in AI models. Learn how this revolutionary approach is enabling faster innovation, reducing biases, and paving the way for more robust AI systems.
Accelerating AI Innovation: The Role of Auto-Generated Data in Machine Learning
The domain of artificial intelligence and machine learning continues to evolve at lightning speed. As advancements grow, so does the requirement for massive amounts of high-quality data to train machine learning models effectively. Yet, acquiring labeled datasets through traditional means can be both time-demanding and resource-intensive.
This is where the concept of auto-generated data enters the picture, offering a powerful alternative to streamlining AI innovations.
Understanding Auto-Generated Data
Auto-generated data refers to the methodologies employed to automatically create datasets that replicate real-world data distribution without the need for manual data gathering or labeling. These advanced techniques leverage synthetic data generation, data augmentation, and adversarial networks to produce comprehensive datasets tailored for robust machine learning training.
Synthetic Data Generation
Synthetic data involves creating artificial data that mimics the statistical properties of original datasets. This process can produce limitless data variations without the constraints associated with confidential or sensitive information. By using generative models, such as Generative Adversarial Networks (GANs), researchers can generate data with high authenticity and greater variance.
Data Augmentation
Data augmentation techniques further extend the variety and quantity of training datasets by methodically altering existing data examples. These modifications include transformations, rotations, noise insertion, and flipping, which help model robustness by enabling exposure to diverse data scenarios.
Adversarial as Data
Adversarial examples are inputs crafted with slight perturbations designed to confuse machine learning models. Although typically seen as a threat to model reliability, adversarial examples paradoxically aid in robust model training by representing challenging data points the models must learn to interpret correctly.
Impact on Machine Learning Efficiency
By implementing auto-generated data techniques, organizations and researchers can:
-
Enhance Data Diversity: Auto-generated datasets offer extensive diversity, improving model generalization across varied applications.
-
Accelerate Model Training: With an abundance of tailored data, model training processes are expedited, cutting down time-to-production significantly.
-
Reduce Data Biases: Introducing synthetic variations reduces potential training biases, which in turn leads to fairer AI solutions.
-
Cost-Effective Data Analytics: By minimizing the need for expensive or tedious data labeling, organizations can reallocate resources to focus on innovation and experimental approaches.
Case Studies in Auto-Generated Data
Healthcare Diagnosis Acceleration
In healthcare, auto-generated data has revolutionized patient diagnostics. By simulating rare disease examples, neural networks now achieve better diagnostic capabilities, allowing earlier disease detection despite limited initial data.
Autonomous Driving Viability
Self-driving car technologies benefit immensely from auto-generated environments. These simulations offer diverse driving scenarios, helping models adapt to different road conditions and improve safety measures for real-time applications.
Financial Sector Innovation
Auto-generated transactional data has enabled financial institutions to simulate anomalies and fraudulent activities, bolstering fraud detection systems with minimal risk to actual assets.
Overcoming Challenges and Ethical Considerations
While auto-generated data fosters machine learning advancements, challenges inevitably arise.
-
Authenticity Verification: Ensuring synthetic data's authenticity demands rigorous generation and validation processes to maintain model reliability.
-
Security Implications: Malicious entities might exploit auto-generated data techniques for harmful purposes unless properly regulated.
-
Ethical Dilemmas: Managing transparency and ethical accountability in synthetic data deployments requires diligent oversight to meet societal standards and legal compliance.
Conclusion
The integration of auto-generated data into AI ecosystems signifies a pivotal shift that dramatically enhances machine learning processes' efficiency, accuracy, and reach. As researchers continue to innovate, the future promises rapid advancements uncovering new horizons for AI applications underscored by robust, machine-generated datasets.
Embracing these transformative practices not only redefines what's possible with AI but also reaffirms the endless potential of machine learning in solving real-world challenges.